Deep learning mixed model-based steady state visual evoked potential classification method
A steady-state visual evoked, hybrid model technology, applied in mechanical mode conversion, electrical digital data processing, character and pattern recognition, etc., can solve steady-state evoked EEG classification difficulties, single feature selection, steady-state visual evoked potential distortion And other issues
- Summary
- Abstract
- Description
- Claims
- Application Information
AI Technical Summary
Problems solved by technology
Method used
Image
Examples
Embodiment Construction
[0095] The present invention will be further described below in conjunction with specific embodiments.
[0096] see figure 1 As shown, the steady-state visually induced EEG classification method based on the deep learning hybrid model described in this embodiment includes the following steps:
[0097] 1) In the stage of EEG signal acquisition
[0098] 1.1) Use the LCD display as the stimulus source to determine the flicker frequency;
[0099] 1.2) Select the electrode channel for collecting EEG to ensure that the electrode channel distributed in the posterior region of the occipital lobe can collect good EEG signals;
[0100] 1.3) Experiments were carried out on multiple different subjects, and a steady-state visual EEG signal database was collected;
[0101] 2) Feature training stage of convolutional neural network
[0102] 2.1) Based on the collected EEG signal database, the EEG data of short time series is used as the input of the convolutional neural network;
[0103] 2...
PUM
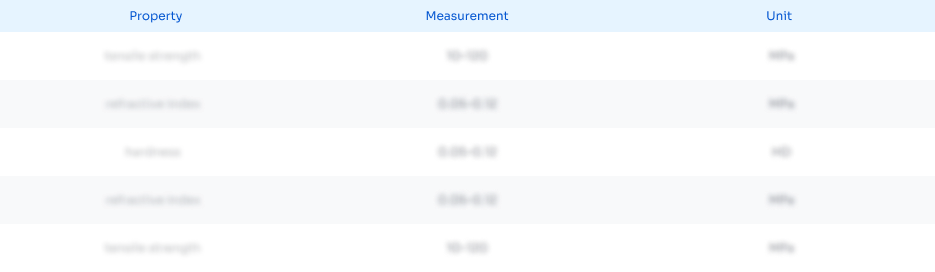
Abstract
Description
Claims
Application Information
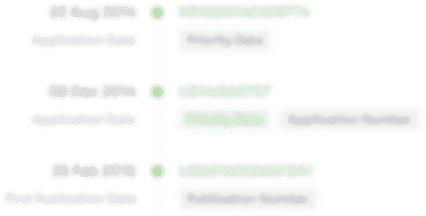
- R&D Engineer
- R&D Manager
- IP Professional
- Industry Leading Data Capabilities
- Powerful AI technology
- Patent DNA Extraction
Browse by: Latest US Patents, China's latest patents, Technical Efficacy Thesaurus, Application Domain, Technology Topic.
© 2024 PatSnap. All rights reserved.Legal|Privacy policy|Modern Slavery Act Transparency Statement|Sitemap