High-spectrum image classification method based on combined loss enhanced network
A technology of hyperspectral image and enhanced network, applied in the field of intelligent image processing, can solve the problems of invariance and poor discrimination of hyperspectral image features, increase in the number of training samples, dimensional disaster, etc., so as to enhance the supervised learning process and reduce the complexity. , the effect of weakening the dependence
- Summary
- Abstract
- Description
- Claims
- Application Information
AI Technical Summary
Problems solved by technology
Method used
Image
Examples
Embodiment
[0024] refer to figure 1 , a hyperspectral image classification method based on a joint loss enhanced network, including the following steps:
[0025] 1) PCA dimensionality reduction: In order to avoid the disaster of dimensionality caused by high-dimensional spectral information, PCA dimensionality reduction is performed on the original hyperspectral image in the spectral dimension, and the compressed spectral dimension is d, in this case d=30 , this step will lose part of the spectral information, but the spatial information of the image will not be affected;
[0026] 2) Spatial domain block extraction: In the hyperspectral image after dimensionality reduction, select the size of the nth pixel of the pre-classification as a w×w×d neighborhood block P n , the P n As the input of the joint loss enhancement network, w×w×d is 21×21×30 in this example;
[0027] 3) Encoding path feature extraction: the encoding channel composed of convolutional layer and pooling layer pairs the...
PUM
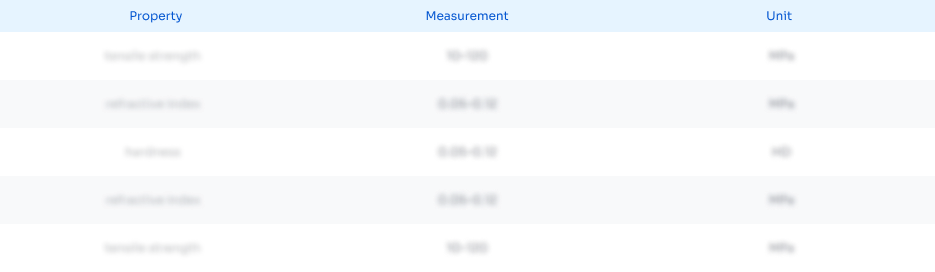
Abstract
Description
Claims
Application Information
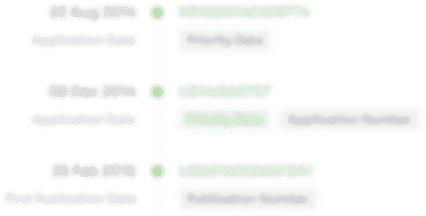
- R&D
- Intellectual Property
- Life Sciences
- Materials
- Tech Scout
- Unparalleled Data Quality
- Higher Quality Content
- 60% Fewer Hallucinations
Browse by: Latest US Patents, China's latest patents, Technical Efficacy Thesaurus, Application Domain, Technology Topic, Popular Technical Reports.
© 2025 PatSnap. All rights reserved.Legal|Privacy policy|Modern Slavery Act Transparency Statement|Sitemap|About US| Contact US: help@patsnap.com