GM-HMM (Gaussian Mixture-Hidden Markov Model) driving behavior prediction method based on visual characteristics
A technology of visual characteristics and behavior, applied in the direction of instruments, character and pattern recognition, computer components, etc., can solve problems such as ignoring abnormal line of sight point elimination, influence of model calculation speed and accuracy, and complication of driving behavior research model establishment
- Summary
- Abstract
- Description
- Claims
- Application Information
AI Technical Summary
Problems solved by technology
Method used
Image
Examples
Embodiment
[0136] The 458 sets of driver's visual representation parameter sequences collected under simulated high-speed conditions, including 102 sets of car-following, 125 sets of left lane changing, 122 sets of right lane changing, and 109 sets of overtaking, were imported into the established GM-HMM driving behavior prediction model Among them, the reliability test results of the GM-HMM driving behavior prediction model are as follows: Image 6 As shown, from the experimental results, it can be seen that when driving on a high-speed road, a GM-HMM prediction driving behavior method based on visual characteristics of the present invention has an accuracy rate of more than 85%, and can more accurately predict the driver's operation behavior. It shows that a GM-HMM method for predicting driving behavior based on visual features is feasible and practical for vehicles driving on highways.
PUM
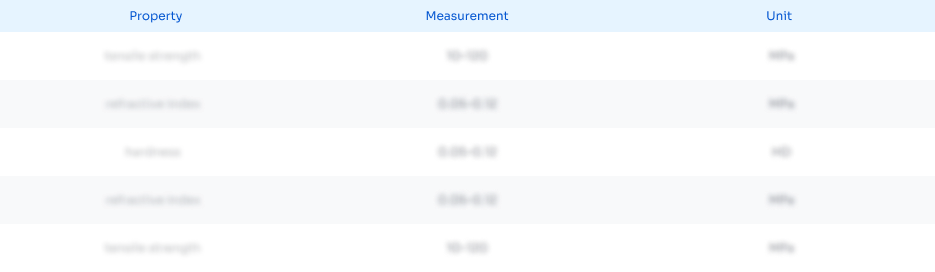
Abstract
Description
Claims
Application Information
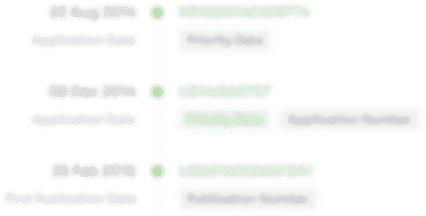
- R&D Engineer
- R&D Manager
- IP Professional
- Industry Leading Data Capabilities
- Powerful AI technology
- Patent DNA Extraction
Browse by: Latest US Patents, China's latest patents, Technical Efficacy Thesaurus, Application Domain, Technology Topic, Popular Technical Reports.
© 2024 PatSnap. All rights reserved.Legal|Privacy policy|Modern Slavery Act Transparency Statement|Sitemap|About US| Contact US: help@patsnap.com