Radar radiation source recognition method based on deep learning strategy and multitask learning strategy
A multi-task learning and deep learning technology, which is applied in neural learning methods, character and pattern recognition, pattern recognition in signals, etc., can solve problems such as poor robustness and poor model generalization performance, and achieve accurate recognition and prevent network The effect of overfitting
- Summary
- Abstract
- Description
- Claims
- Application Information
AI Technical Summary
Problems solved by technology
Method used
Image
Examples
Embodiment Construction
[0033] Below in conjunction with accompanying drawing, technical scheme and effect of the present invention are further described:
[0034] refer to figure 1 , the implementation steps of the present invention are as follows:
[0035] Step 1, data preprocessing is performed on the radar emitter signal.
[0036] Radar emitter signals are often affected by factors such as the environment and receiving equipment in the process of propagation, acquisition and conversion, and the signal is more seriously interfered. The noise reduction of the obtained radar emitter signal is an important part of radar emitter signal identification. . Secondly, because wavelet transform has the characteristics of low entropy, multi-resolution, decorrelation and flexible basis functions, the method of using wavelet transform to realize signal-noise separation in wavelet domain has been widely used. Finally, in order to make the noise reduction The energy of the radar emitter signal is on the same ...
PUM
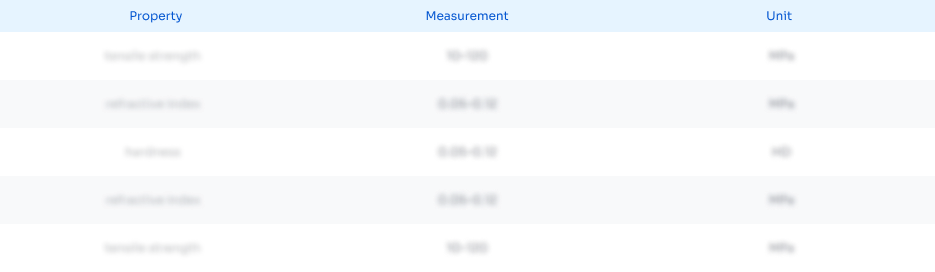
Abstract
Description
Claims
Application Information
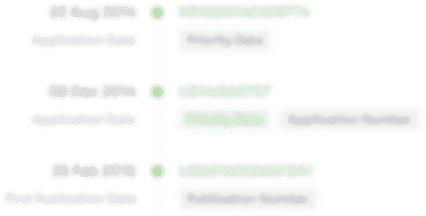
- Generate Ideas
- Intellectual Property
- Life Sciences
- Materials
- Tech Scout
- Unparalleled Data Quality
- Higher Quality Content
- 60% Fewer Hallucinations
Browse by: Latest US Patents, China's latest patents, Technical Efficacy Thesaurus, Application Domain, Technology Topic, Popular Technical Reports.
© 2025 PatSnap. All rights reserved.Legal|Privacy policy|Modern Slavery Act Transparency Statement|Sitemap|About US| Contact US: help@patsnap.com