Dynamic texture recognition method based on two bipartite graph
A technology of dynamic texture and recognition method, applied in image data processing, character and pattern recognition, image analysis and other directions, can solve problems affecting optical flow calculation, loss of sports field, etc., and achieve the effect of simple segmentation algorithm and good effect.
- Summary
- Abstract
- Description
- Claims
- Application Information
AI Technical Summary
Problems solved by technology
Method used
Image
Examples
Embodiment 1
[0074] In this embodiment, the dynamic texture segmentation database is 100 dynamic texture videos obtained through artificial synthesis, in which only two kinds of dynamic textures appear. image 3 The middle left column gives examples of texture images in the database. The results obtained by segmentation with chaotic eigenvectors are shown in image 3 middle column. The results obtained by segmenting the pixel time series are shown in image 3 Center right column. It can be seen from the experimental results that the results obtained by using chaotic eigenvector segmentation are better than those obtained by using pixel time series. Shown in the figure is the preliminary result of segmentation, and the noise in some places can be improved by the morphology in image processing.
Embodiment 2
[0076] This embodiment is synthesized by adding flames on the water surface. The dynamic flame constantly changes position. Figure 4 The segmentation results are given in . On the left is the picture in the original video, in the middle is the segmentation result obtained by using the chaotic feature vector as the feature, and on the right is the segmentation result obtained by using the pixel time series as the feature.
Embodiment 3
[0078] In this implementation, it is dense scene data. Intensive scenes exist widely in real life, especially large gatherings. It is quite difficult to track, recognize and understand dense scenes, but it has important practical application value. We do a preliminary study of this problem by segmenting dense scenes. Figure 5 The middle left column gives examples of texture images in the database. The results obtained by segmentation with chaotic eigenvectors are shown in Figure 5 middle column. The results obtained by segmenting the pixel time series are shown in Figure 5 Center right column. Figure 5 (a) shows the crowd moving in a circle in Mecca. The crowd in the video has two movement directions, some moving clockwise and some moving counterclockwise. The motion of the two parts is obtained by a segmentation algorithm. Figure 5 (b) shows dense traffic flow. Figure 5 Shown in (c) is the flow of traffic traveling on the highway. Figure 5A video of dense crow...
PUM
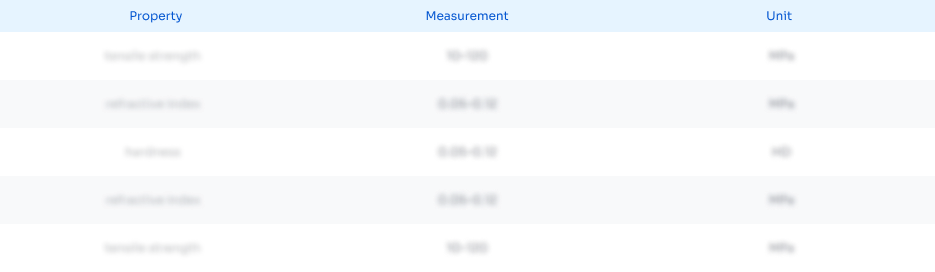
Abstract
Description
Claims
Application Information
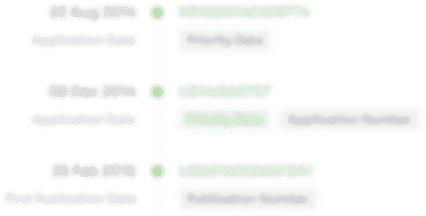
- R&D Engineer
- R&D Manager
- IP Professional
- Industry Leading Data Capabilities
- Powerful AI technology
- Patent DNA Extraction
Browse by: Latest US Patents, China's latest patents, Technical Efficacy Thesaurus, Application Domain, Technology Topic, Popular Technical Reports.
© 2024 PatSnap. All rights reserved.Legal|Privacy policy|Modern Slavery Act Transparency Statement|Sitemap|About US| Contact US: help@patsnap.com