Method for predicting solar power generation amount based on adaptive learning hybrid model
A technology of self-adaptive learning and hybrid model, applied in neural learning methods, forecasting, biological neural network models, etc., can solve problems such as limiting the efficiency and reliability of microgrid energy management, random fluctuations of solar energy, and inability to meet energy management requirements , to achieve satisfactory prediction results, improve prediction accuracy, and efficient energy management
- Summary
- Abstract
- Description
- Claims
- Application Information
AI Technical Summary
Problems solved by technology
Method used
Image
Examples
Embodiment Construction
[0034] Below in conjunction with accompanying drawing, the technical scheme of invention is described in detail:
[0035] figure 1 It is a flow chart of the steps of the prediction method of the present invention; figure 2 It is a schematic diagram of the structural composition of the self-adaptive learning hybrid model of the present invention, which is composed of a time-varying multivariate linear model and a learning model.
[0036] Obtain the future t time and its time bandwidth according to the weather forecast A set of weather numbers in the range
[0037] Obtain a time-varying multivariate linear model through step (2):
[0038]
[0039] Training Sample Dataset Using Meteorological Variables According to the above model to get a preliminary prediction data set Then get the preliminary prediction data set The prediction error data set of
[0040]
[0041] in, and The calculation steps are as follows:
[0042] Set a value for the bandwidth h, a...
PUM
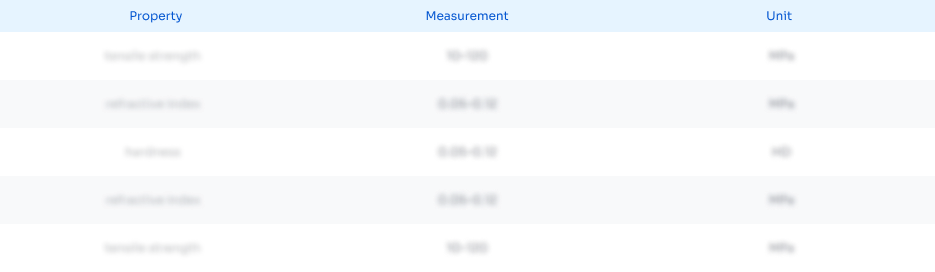
Abstract
Description
Claims
Application Information
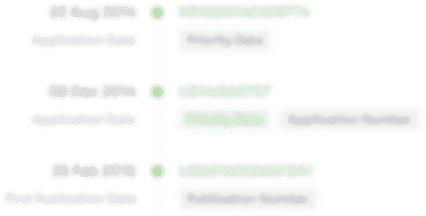
- R&D
- Intellectual Property
- Life Sciences
- Materials
- Tech Scout
- Unparalleled Data Quality
- Higher Quality Content
- 60% Fewer Hallucinations
Browse by: Latest US Patents, China's latest patents, Technical Efficacy Thesaurus, Application Domain, Technology Topic, Popular Technical Reports.
© 2025 PatSnap. All rights reserved.Legal|Privacy policy|Modern Slavery Act Transparency Statement|Sitemap|About US| Contact US: help@patsnap.com