Multi-feature-learning-based adversarial network training method
A network training, multi-feature technology, applied in the field of deep learning neural network, can solve problems such as low efficiency of network learning and training, and achieve the effect of improving efficiency
- Summary
- Abstract
- Description
- Claims
- Application Information
AI Technical Summary
Problems solved by technology
Method used
Image
Examples
Embodiment
[0026] This embodiment discloses a multi-feature learning confrontation network training method, which specifically includes the following steps:
[0027] Step S1. Construct a deep convolutional generative confrontation network DCGAN model. The generator generates images and inputs them to the discriminator for network training.
[0028] Step S2, constructing multiple convolution kernels for the discriminator;
[0029] Different convolution kernels are reflected in different matrix values and different numbers of rows and columns.
[0030] Construct multiple convolution kernels. In the process of processing images, different convolution kernels mean that different features of generated images can be learned during network training.
[0031] In the traditional confrontation network model, the discriminator can only perform the convolution of the next layer based on the result of the convolution of the previous layer. In this case, the features learned by the discriminator a...
PUM
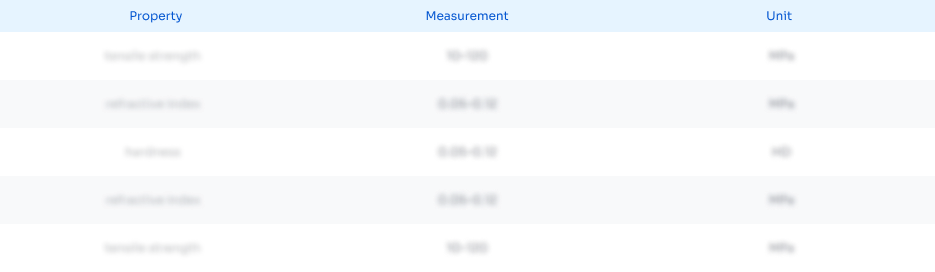
Abstract
Description
Claims
Application Information
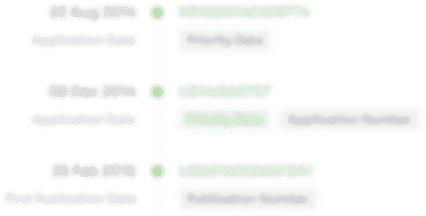
- R&D Engineer
- R&D Manager
- IP Professional
- Industry Leading Data Capabilities
- Powerful AI technology
- Patent DNA Extraction
Browse by: Latest US Patents, China's latest patents, Technical Efficacy Thesaurus, Application Domain, Technology Topic, Popular Technical Reports.
© 2024 PatSnap. All rights reserved.Legal|Privacy policy|Modern Slavery Act Transparency Statement|Sitemap|About US| Contact US: help@patsnap.com