Multi-category multi-view data generation method for generating confrontation network based on depth convolution
A technology of data generation and deep convolution, which is applied in the fields of deep learning and image processing, can solve the problems of high acquisition cost and small data volume, and achieve the effect of reducing the number of nodes, eliminating interference, and connecting closely
- Summary
- Abstract
- Description
- Claims
- Application Information
AI Technical Summary
Problems solved by technology
Method used
Image
Examples
Embodiment Construction
[0038] The present invention will be further described below with reference to the accompanying drawings and taking pearl data as an example.
[0039] refer to Figure 1 to Figure 5 , a multi-category multi-view data generation method based on a deep convolutional generative adversarial network, comprising the following steps:
[0040] Starting with a batch of pearl five-view data divided into 7 categories,
[0041] Step 1: Center Cut:
[0042] The five views consist of a top view and four side views, each with an original size of 300*300*3, including a pearl image in the center and a black background. According to experiments, cropping the picture to 250*250*3 will not affect the pearl image, and can save nearly 30% of pixels.
[0043] Step 2: Multi-view superposition:
[0044] Superimpose the five pearl maps according to the channel dimension, such as image 3 , forming a multi-dimensional matrix of 250*250*15, as a piece of image data, a total of 10,500 pieces of image...
PUM
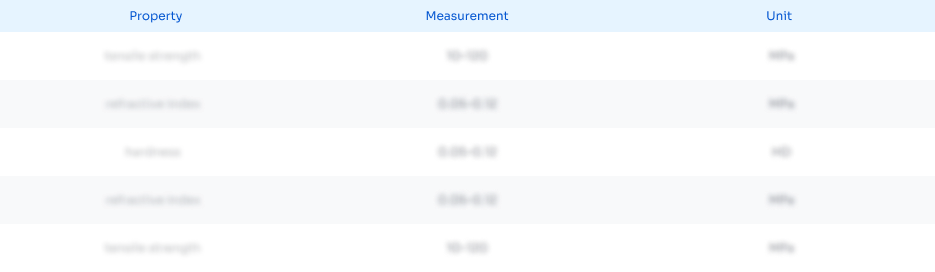
Abstract
Description
Claims
Application Information
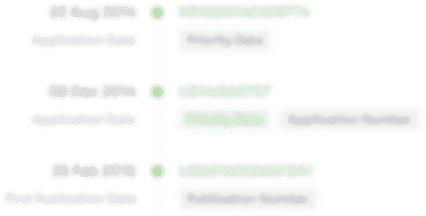
- R&D
- Intellectual Property
- Life Sciences
- Materials
- Tech Scout
- Unparalleled Data Quality
- Higher Quality Content
- 60% Fewer Hallucinations
Browse by: Latest US Patents, China's latest patents, Technical Efficacy Thesaurus, Application Domain, Technology Topic, Popular Technical Reports.
© 2025 PatSnap. All rights reserved.Legal|Privacy policy|Modern Slavery Act Transparency Statement|Sitemap|About US| Contact US: help@patsnap.com