Fabric defect detection method based on multi-feature matrix low-rank decomposition
A low-rank decomposition and detection method technology, applied in image analysis, image data processing, instruments, etc., can solve the problem of low detection accuracy
- Summary
- Abstract
- Description
- Claims
- Application Information
AI Technical Summary
Problems solved by technology
Method used
Image
Examples
specific Embodiment
[0103] In a specific embodiment, randomly select several types of common defect images (including wrong weft, broken warp, jumping flowers, damage, broken weft, etc.) from the fabric image library, and the size of the pictures is 256pixel * 256pixel, such as image 3 As shown in (a), it is the defect image from top to bottom. The image block size is selected as 16pixel×16pixel. The selected feature dimension d is 128, the balance factor λ is 0.75, and the number of channels H=8. right image 3 The saliency map generated by the saliency model based on the low-level feature wavelet transform in (a) is shown as image 3 As shown in (b), it can be seen from the figure that this method can hardly detect defect areas effectively for complex pattern images. right image 3 The saliency map generated based on the histogram of oriented gradients and the low-rank decomposition model in (a) is as follows image 3 As shown in (c), it can be seen from the figure that the method has ach...
PUM
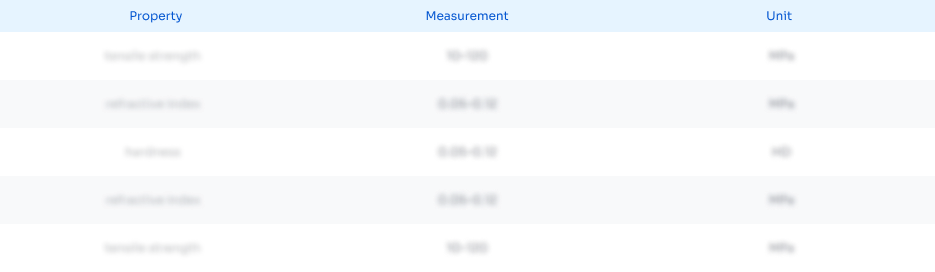
Abstract
Description
Claims
Application Information
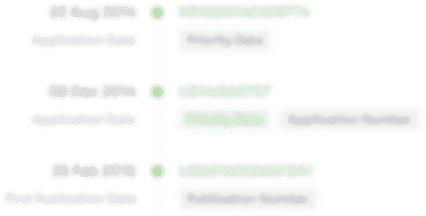
- R&D Engineer
- R&D Manager
- IP Professional
- Industry Leading Data Capabilities
- Powerful AI technology
- Patent DNA Extraction
Browse by: Latest US Patents, China's latest patents, Technical Efficacy Thesaurus, Application Domain, Technology Topic, Popular Technical Reports.
© 2024 PatSnap. All rights reserved.Legal|Privacy policy|Modern Slavery Act Transparency Statement|Sitemap|About US| Contact US: help@patsnap.com