Neural machine translation method based on Multi-BiRNN encoding
A machine translation and encoding technology, applied in the field of natural language translation, to achieve the effect of improving performance
- Summary
- Abstract
- Description
- Claims
- Application Information
AI Technical Summary
Problems solved by technology
Method used
Image
Examples
Embodiment Construction
[0027] The present invention will be further elaborated below in conjunction with the accompanying drawings of the description.
[0028] A kind of neural machine translation method based on Multi-BiRNN coding of the present invention comprises the following steps:
[0029] 1) The Multi-BiRNN encoding method is adopted at the encoder end, that is, on the basis of the source language sentence as the input sequence, one or more sets of BiRNN are added to encode other related input sequences;
[0030] 2) Neural machine translation based on Multi-BiRNN coding. In the source encoding process, the source language sentence sequence and its dependent syntax tree are considered at the same time, and the serialization results of the syntax tree are obtained through two different traversal methods, which are different from the source language sentence sequence. Sequence together as the input of Multi-BiRNN encoding;
[0031] 3) At the output end of each group of BiRNN, each word is forme...
PUM
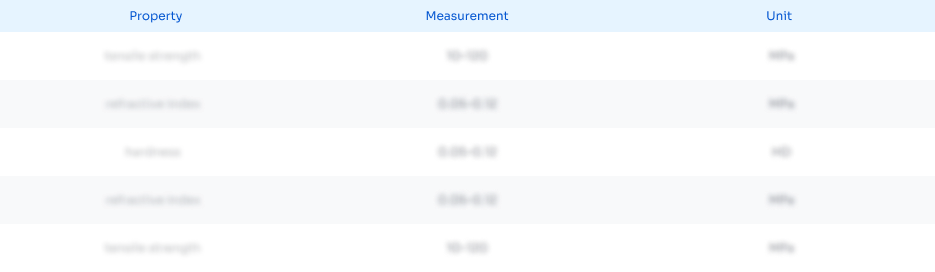
Abstract
Description
Claims
Application Information
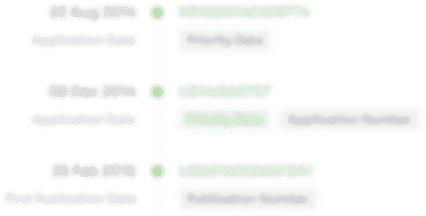
- R&D Engineer
- R&D Manager
- IP Professional
- Industry Leading Data Capabilities
- Powerful AI technology
- Patent DNA Extraction
Browse by: Latest US Patents, China's latest patents, Technical Efficacy Thesaurus, Application Domain, Technology Topic.
© 2024 PatSnap. All rights reserved.Legal|Privacy policy|Modern Slavery Act Transparency Statement|Sitemap