A Behavior Recognition Method Based on Motion History Images and Convolutional Neural Networks
A convolutional neural network, motion history image technology, applied in neural learning methods, biological neural network models, image analysis, etc., can solve the problems of low behavior recognition accuracy, slow algorithm speed, etc., and achieve simple and efficient feature extraction. Accuracy, the effect of increasing speed
- Summary
- Abstract
- Description
- Claims
- Application Information
AI Technical Summary
Problems solved by technology
Method used
Image
Examples
Embodiment Construction
[0054] In order to make the object, technical solution and advantages of the present invention clearer, the present invention will be further described in detail below in conjunction with the accompanying drawings and embodiments. It should be understood that the specific embodiments described here are only used to explain the present invention, not to limit the present invention.
[0055] Such as figure 1 As shown, the behavior recognition method based on motion history images and convolutional neural network in the embodiment of the present invention comprises the following steps:
[0056] S1. Obtain the input original video image, and process it through the behavior sequence feature extraction method based on the motion history image: first extract the foreground in the original video image through the frame difference algorithm, and then generate the global motion history image from the foreground within a period of time , using the minimum external rectangle principle to...
PUM
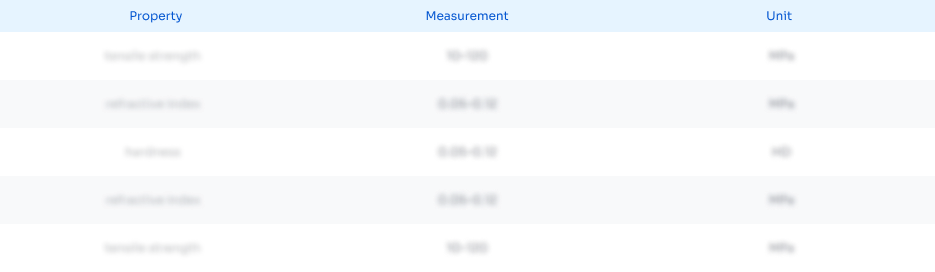
Abstract
Description
Claims
Application Information
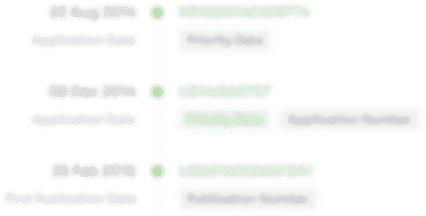
- R&D Engineer
- R&D Manager
- IP Professional
- Industry Leading Data Capabilities
- Powerful AI technology
- Patent DNA Extraction
Browse by: Latest US Patents, China's latest patents, Technical Efficacy Thesaurus, Application Domain, Technology Topic.
© 2024 PatSnap. All rights reserved.Legal|Privacy policy|Modern Slavery Act Transparency Statement|Sitemap