Rice detection and classification method based on deep multi-view feature
A classification method and multi-view technology, applied in character and pattern recognition, instruments, biological neural network models, etc., can solve the problems of low registration point accuracy, large error in manual detection, and error in roughness, etc., to achieve improvement Insufficient rice feature extraction, improvement of accuracy and efficiency, and the effect of improving collection efficiency
- Summary
- Abstract
- Description
- Claims
- Application Information
AI Technical Summary
Problems solved by technology
Method used
Image
Examples
Embodiment Construction
[0045] A method used for rice detection and classification, the method of the present invention is a deep learning method.
[0046] A model framework used for rice detection and classification, the model framework of the present invention is a multi-layer convolutional neural network.
[0047] A rice detection and classification method based on deep multi-viewpoint features, the method includes the following steps: 1) division of data sets: use cross-validation method to divide the data set of rice falling images to obtain training sets and test sets; 2) network Model construction: use the method of deep learning to extract the features of the input image, and use stochastic gradient descent to continuously update the model parameters; 3) model analysis and performance evaluation: input the test data into the trained rice classifier, pass The detection and classification results of rice are obtained by comparing the probability prediction value with the classification threshol...
PUM
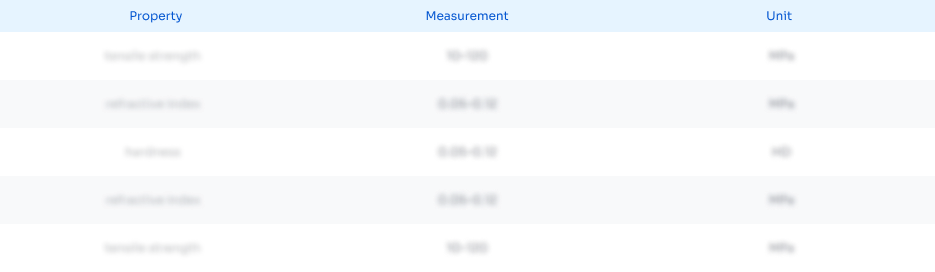
Abstract
Description
Claims
Application Information
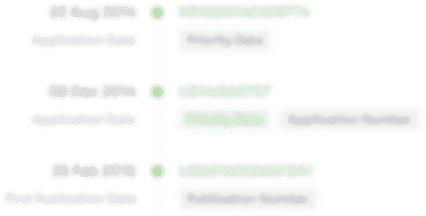
- R&D Engineer
- R&D Manager
- IP Professional
- Industry Leading Data Capabilities
- Powerful AI technology
- Patent DNA Extraction
Browse by: Latest US Patents, China's latest patents, Technical Efficacy Thesaurus, Application Domain, Technology Topic, Popular Technical Reports.
© 2024 PatSnap. All rights reserved.Legal|Privacy policy|Modern Slavery Act Transparency Statement|Sitemap|About US| Contact US: help@patsnap.com