Semantic segmentation method based on multi-scale convolutional neural networks (CNNs)
A convolutional neural network, multi-scale segmentation technology, applied in the computer field, can solve the problems of ignoring statistical characteristics between modes, easy to produce salt and pepper effect, blurred object boundaries, etc.
- Summary
- Abstract
- Description
- Claims
- Application Information
AI Technical Summary
Problems solved by technology
Method used
Image
Examples
Embodiment Construction
[0037] The following will clearly and completely describe the technical solutions in the embodiments of the present invention with reference to the accompanying drawings in the embodiments of the present invention. Obviously, the described embodiments are only some of the embodiments of the present invention, not all of them. Based on the embodiments of the present invention, all other embodiments obtained by persons of ordinary skill in the art without creative efforts fall within the protection scope of the present invention.
[0038] The present invention involves multi-scale CNN-based classification and post-processing of multi-scale segmentation. First, segment-to-end multi-scale CNN is used to fuse and classify high-resolution images and LiDAR point cloud data, and then multi-scale segmentation is used to The method extracts the object boundary and optimizes the classification result, specifically figure 1 The flow chart of the semantic segmentation method based on the m...
PUM
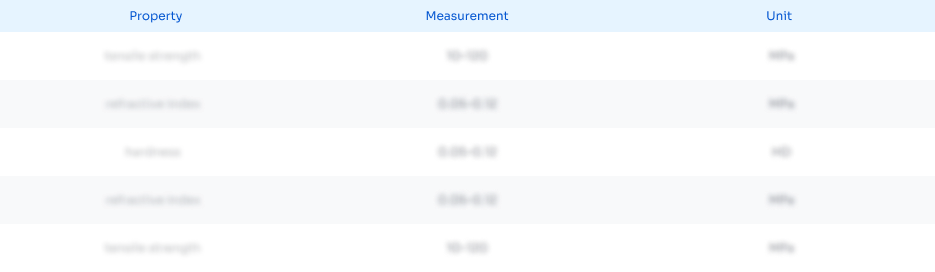
Abstract
Description
Claims
Application Information
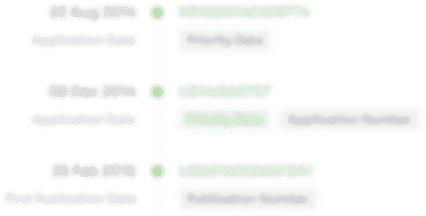
- Generate Ideas
- Intellectual Property
- Life Sciences
- Materials
- Tech Scout
- Unparalleled Data Quality
- Higher Quality Content
- 60% Fewer Hallucinations
Browse by: Latest US Patents, China's latest patents, Technical Efficacy Thesaurus, Application Domain, Technology Topic, Popular Technical Reports.
© 2025 PatSnap. All rights reserved.Legal|Privacy policy|Modern Slavery Act Transparency Statement|Sitemap|About US| Contact US: help@patsnap.com