Method for recognizing early fault of bearing based on long and short-term memory recurrent neural network
A technology of cyclic neural network and long-short-term memory, applied in biological neural network models, neural architecture, mechanical bearing testing, etc., can solve the problems of insufficient utilization of historical signals in the degradation process, lack of characteristic quantities of bearing degradation trends, etc., to avoid spectrum Analytical methods, the effect of accurate identification
- Summary
- Abstract
- Description
- Claims
- Application Information
AI Technical Summary
Problems solved by technology
Method used
Image
Examples
Embodiment Construction
[0069] The technical solution of the present invention will be described in further detail below in combination with specific embodiments according to the drawings in the description.
[0070] like figure 1 a~ figure 1 As shown in b, a bearing early fault recognition method based on long-short-term memory recurrent neural network, including the following steps:
[0071] S1. Collect the vibration signal of the whole life of the bearing, and extract the commonly used time domain features after the vibration signal is preprocessed;
[0072] S2. Select the waveform factor in the time domain feature to construct the waveform entropy feature, and use the square demodulation method in the spectrum analysis to verify the validity of the waveform entropy;
[0073] S3. Construct a feature data set by using time-domain features and entropy features, and select a normal data set and a deep fault data set through the judgment of time-domain signal and spectrum analysis;
[0074] S4. The...
PUM
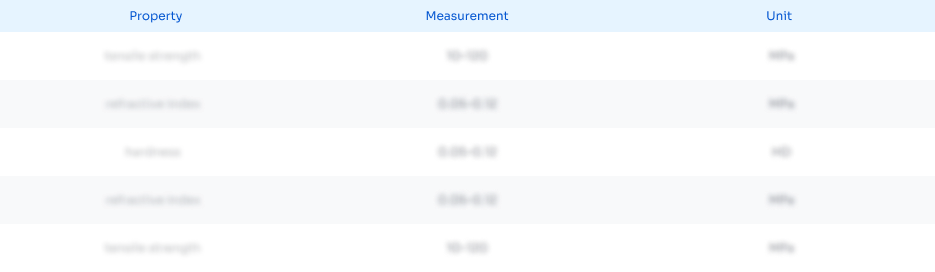
Abstract
Description
Claims
Application Information
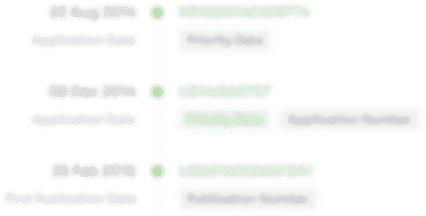
- R&D Engineer
- R&D Manager
- IP Professional
- Industry Leading Data Capabilities
- Powerful AI technology
- Patent DNA Extraction
Browse by: Latest US Patents, China's latest patents, Technical Efficacy Thesaurus, Application Domain, Technology Topic, Popular Technical Reports.
© 2024 PatSnap. All rights reserved.Legal|Privacy policy|Modern Slavery Act Transparency Statement|Sitemap|About US| Contact US: help@patsnap.com