Image denoising method combining Bayes layered learning with space-spectrum combined priori
A technology of joint prior and image noise reduction, applied in the information field, it can solve the problems of poor noise reduction, inability to directly use and restore noiseless data, and ignoring local structural differences.
- Summary
- Abstract
- Description
- Claims
- Application Information
AI Technical Summary
Problems solved by technology
Method used
Image
Examples
Embodiment Construction
[0080] The present invention will be described in detail below in conjunction with the drawings and embodiments, but the protection scope of the present invention is not limited to the embodiments.
[0081] The present invention improves the existing hyperspectral image denoising method, and proposes a hyperspectral image denoising method combining Bayesian layered learning and space-spectrum joint prior. refer to figure 1 , the present invention mines the spatial spectral correlation and non-local self-similarity of hyperspectral images through collaborative block learning, and can effectively express pixels in edges and regions with large differences; uses low-rank decomposition to learn and represent collaborative block data The low-rank characteristics in the spatial spectral domain realize the learning of data structure information; and use the MOG noise modulus combined with the Dirichlet process to simulate the noise characteristics of hyperspectral images to achieve th...
PUM
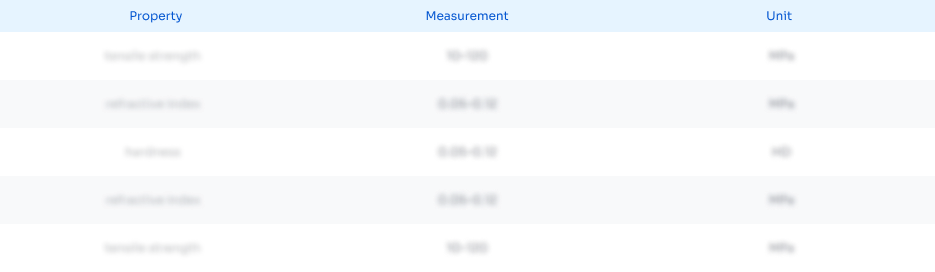
Abstract
Description
Claims
Application Information
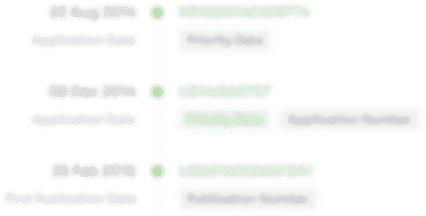
- R&D Engineer
- R&D Manager
- IP Professional
- Industry Leading Data Capabilities
- Powerful AI technology
- Patent DNA Extraction
Browse by: Latest US Patents, China's latest patents, Technical Efficacy Thesaurus, Application Domain, Technology Topic, Popular Technical Reports.
© 2024 PatSnap. All rights reserved.Legal|Privacy policy|Modern Slavery Act Transparency Statement|Sitemap|About US| Contact US: help@patsnap.com