Three-dimensional convolution and Faster RCNN-based video action detection method
A three-dimensional convolution and motion detection technology, applied in the field of image processing, can solve the problems of synchronous positioning and lack of spatial annotation information, and achieve the effect of motion positioning and excellent performance.
- Summary
- Abstract
- Description
- Claims
- Application Information
AI Technical Summary
Problems solved by technology
Method used
Image
Examples
Embodiment 1
[0093] In the present invention, NVIDIA GPU is used as the computing platform, CUDA is used as the GPU accelerator, and Caffe is selected as the CNN framework.
[0094] S1 data preparation:
[0095] The ActivityNet 1.3 dataset is used in this experiment. The ActivityNet dataset consists only of untrimmed videos with 200 different types of activities, including 10024 videos in the training set, 4926 videos in the validation set and 5044 videos in the test set. Compared to THUMOS14, this is a large dataset, both in terms of the number of activity categories involved and the number of videos.
[0096] Step 1.1: Download the ActivityNet 1.3 dataset from http: / / activity-net.org / download.html to the local.
[0097] Step 1.2: Convert the downloaded video into images according to 25 frames per second (fps), and the images of different subsets are placed in folders according to the corresponding video names.
[0098] Step 1.3: According to the data augmentation strategy, this experi...
PUM
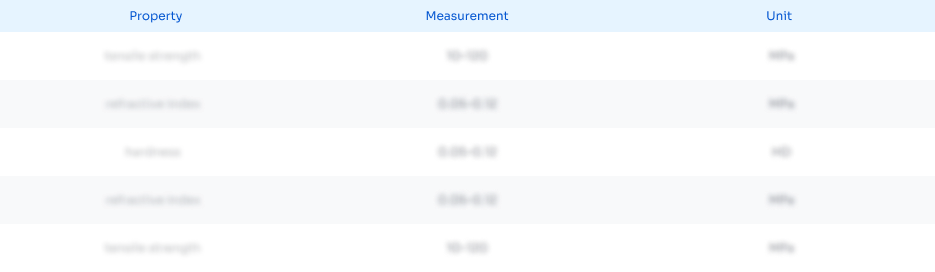
Abstract
Description
Claims
Application Information
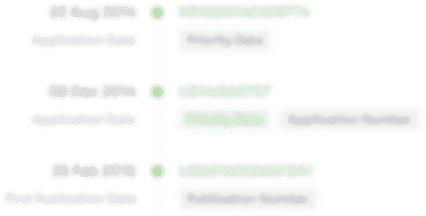
- R&D Engineer
- R&D Manager
- IP Professional
- Industry Leading Data Capabilities
- Powerful AI technology
- Patent DNA Extraction
Browse by: Latest US Patents, China's latest patents, Technical Efficacy Thesaurus, Application Domain, Technology Topic, Popular Technical Reports.
© 2024 PatSnap. All rights reserved.Legal|Privacy policy|Modern Slavery Act Transparency Statement|Sitemap|About US| Contact US: help@patsnap.com