Road scene segmentation method based on full convolutional neural network
A convolutional neural network and convolutional neural network technology, applied in the fields of unmanned driving, image segmentation, target recognition, and target retrieval, can solve the problem that the segmentation accuracy of road signs, vehicles, and pedestrians does not reach the ideal result, and the segmentation effect of complex scenes Poor, difficult to meet practical requirements and other problems, to achieve the effect of solving the problem of road scene segmentation, preventing over-segmentation, and solving the problem of segmentation
- Summary
- Abstract
- Description
- Claims
- Application Information
AI Technical Summary
Problems solved by technology
Method used
Image
Examples
Embodiment Construction
[0034] The technical solution of the present invention will be further described below in conjunction with the accompanying drawings.
[0035] Such as figure 1 As shown, the original data set is processed into a training set through the improved KSW double-threshold segmentation and genetic algorithm, and different weights are selected in the FCN network framework. The FCN-16s model is obtained; the FCN-8s model is obtained by selecting the FCN-16s weight. According to the results of multiple experiments, the effect of the FCN-16s model is the best, and the present invention selects the weight of the FCN-32s to build the FCNN network framework.
[0036] Step 1, put the original road scene image as initial data into the improved KSW (best entropy) two-dimensional threshold and genetic algorithm to obtain the training set of deep learning, and the test set can use the original RGB image. The specific implementation steps include the following steps;
[0037] Step 1a, first set...
PUM
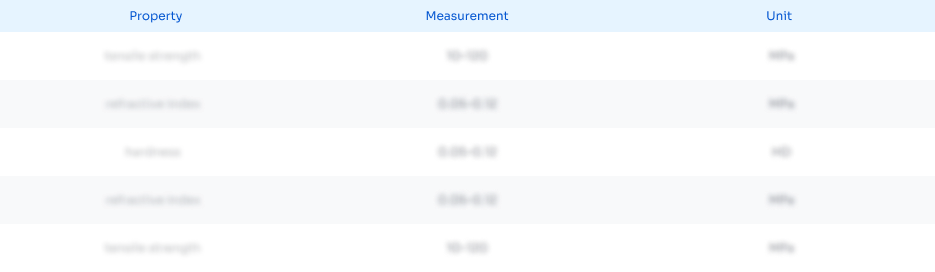
Abstract
Description
Claims
Application Information
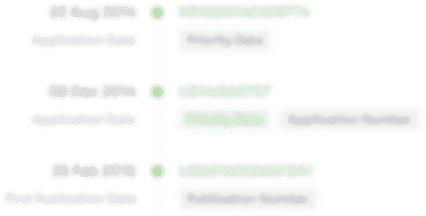
- R&D Engineer
- R&D Manager
- IP Professional
- Industry Leading Data Capabilities
- Powerful AI technology
- Patent DNA Extraction
Browse by: Latest US Patents, China's latest patents, Technical Efficacy Thesaurus, Application Domain, Technology Topic, Popular Technical Reports.
© 2024 PatSnap. All rights reserved.Legal|Privacy policy|Modern Slavery Act Transparency Statement|Sitemap|About US| Contact US: help@patsnap.com