Urban road network travel time estimation method based on adaptive multi-task deep learning
A multi-task learning and travel time technology, applied in the field of urban road network travel time estimation, can solve problems such as difficulty in obtaining observation values and unsatisfactory model training effects, and achieve high estimation accuracy
- Summary
- Abstract
- Description
- Claims
- Application Information
AI Technical Summary
Problems solved by technology
Method used
Image
Examples
Embodiment Construction
[0046] Below in conjunction with accompanying drawing, technical scheme of the present invention is described in further detail:
[0047] Those skilled in the art can understand that, unless otherwise defined, all terms (including technical terms and scientific terms) used herein have the same meaning as commonly understood by one of ordinary skill in the art to which this invention belongs. It should also be understood that terms such as those defined in commonly used dictionaries should be understood to have a meaning consistent with the meaning in the context of the prior art, and will not be interpreted in an idealized or overly formal sense unless defined as herein Explanation.
[0048] refer to figure 1 As shown, this section includes the following five steps. Firstly, the characteristic factors affecting the travel time of road segments are extracted to determine the input of the model. Consider the travel time estimation of all road segments in a time period as a ta...
PUM
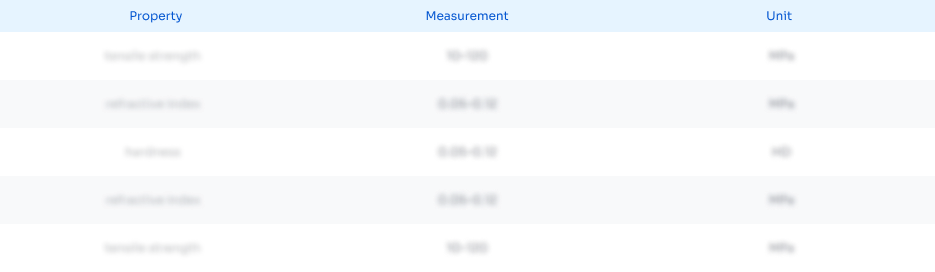
Abstract
Description
Claims
Application Information
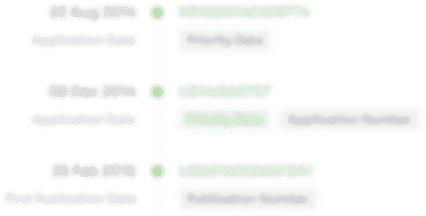
- R&D
- Intellectual Property
- Life Sciences
- Materials
- Tech Scout
- Unparalleled Data Quality
- Higher Quality Content
- 60% Fewer Hallucinations
Browse by: Latest US Patents, China's latest patents, Technical Efficacy Thesaurus, Application Domain, Technology Topic, Popular Technical Reports.
© 2025 PatSnap. All rights reserved.Legal|Privacy policy|Modern Slavery Act Transparency Statement|Sitemap|About US| Contact US: help@patsnap.com