A weak fault diagnosis method for rolling bearings based on wavelet transform and deep learning
A rolling bearing and fault diagnosis technology, which is applied in the testing of mechanical components, testing of machine/structural components, instruments, etc., can solve problems such as rough discrete intervals and affecting fault feature extraction, and achieve strong learning and expression capabilities, fast computing speed, The effect of reducing the impact
- Summary
- Abstract
- Description
- Claims
- Application Information
AI Technical Summary
Problems solved by technology
Method used
Image
Examples
Embodiment Construction
[0028] The technical solution of the present invention will be further described below in conjunction with the accompanying drawings.
[0029] The present invention provides a rolling bearing weak fault diagnosis method based on wavelet transform and deep learning, such as figure 1 As shown, the method includes the following steps:
[0030] Step 1. Data Acquisition
[0031] Prepare 200 rolling bearings of model NSK-6304, of which 50 are normal bearings, 50 are inner ring faulty bearings, 50 are outer ring faulty bearings, and 50 are ball body faulty bearings. The three kinds of faulty bearings were obtained by using an engraving machine to artificially create slight scratches on the inner ring, outer ring and rolling elements of the corresponding bearings, so as to simulate the early faults of rolling bearings. Use the vibration acceleration sensor to collect the vibration acceleration signals of various rolling bearings at a speed of 600r / min at a sampling frequency of 16kH...
PUM
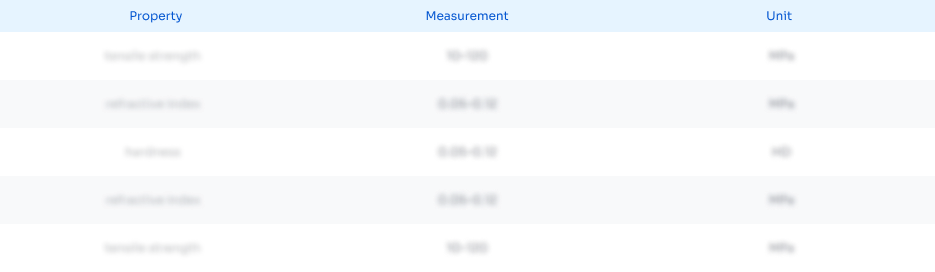
Abstract
Description
Claims
Application Information
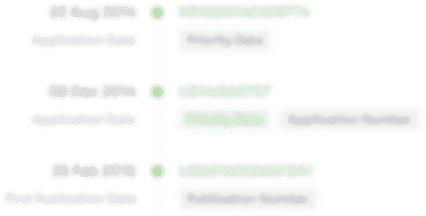
- Generate Ideas
- Intellectual Property
- Life Sciences
- Materials
- Tech Scout
- Unparalleled Data Quality
- Higher Quality Content
- 60% Fewer Hallucinations
Browse by: Latest US Patents, China's latest patents, Technical Efficacy Thesaurus, Application Domain, Technology Topic, Popular Technical Reports.
© 2025 PatSnap. All rights reserved.Legal|Privacy policy|Modern Slavery Act Transparency Statement|Sitemap|About US| Contact US: help@patsnap.com