Road extraction method based on fully convolutional network ensemble learning
A convolutional neural network and road extraction technology, applied in neural learning methods, biological neural network models, neural architectures, etc., can solve problems that do not take into account the unbalanced distribution of roads and backgrounds in remote sensing images, and the spatial consistency of remote sensing images It does not take into account the spatial consistency of remote sensing images, etc., and achieves the effect of improving recall rate, good performance, and improving robustness.
- Summary
- Abstract
- Description
- Claims
- Application Information
AI Technical Summary
Problems solved by technology
Method used
Image
Examples
Embodiment Construction
[0035] The present invention will be further described in detail below in conjunction with the drawings and specific embodiments.
[0036] Reference figure 1 , One A road extraction method based on fully convolutional neural network ensemble learning includes the following steps:
[0037] Step 1) Divide the input remote sensing image to construct a training sample set and a test sample set:
[0038] Acquire M optical remote sensing images with a size of N×N and binary class standard images corresponding to the optical remote sensing images, and use these optical remote sensing images and binary class standard images as sample sets, where N≥64, M≥100 .
[0039] In the existing remote sensing image database, most of the remote sensing image frames are N×N square images. When the fully convolutional neural network performs feature extraction, it will downsample the input image many times, so the size of the input image has a lower limit. The general remote sensing image size is betwe...
PUM
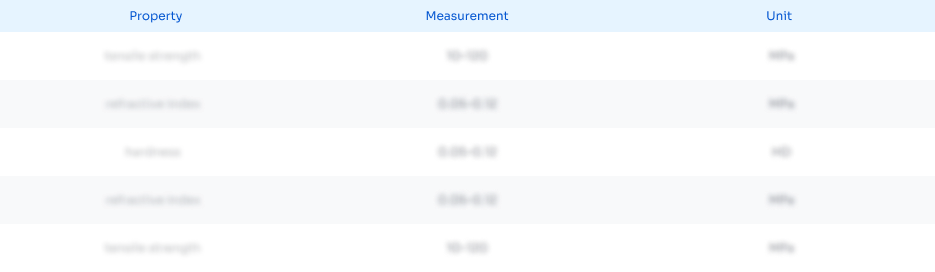
Abstract
Description
Claims
Application Information
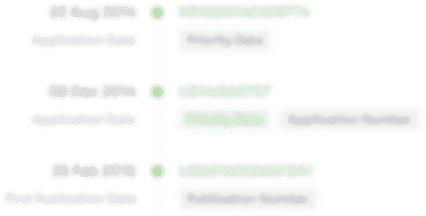
- R&D Engineer
- R&D Manager
- IP Professional
- Industry Leading Data Capabilities
- Powerful AI technology
- Patent DNA Extraction
Browse by: Latest US Patents, China's latest patents, Technical Efficacy Thesaurus, Application Domain, Technology Topic, Popular Technical Reports.
© 2024 PatSnap. All rights reserved.Legal|Privacy policy|Modern Slavery Act Transparency Statement|Sitemap|About US| Contact US: help@patsnap.com