Hyperspectral hyperpixel segmentation method based on principal component weighted false color synthesis and color histogram driving
A technology of false color synthesis and color histogram, applied in image enhancement, image data processing, image analysis, etc., can solve problems such as large data redundancy, difficult real-time image segmentation, and high data dimensionality of hyperspectral images
- Summary
- Abstract
- Description
- Claims
- Application Information
AI Technical Summary
Problems solved by technology
Method used
Image
Examples
specific Embodiment approach 1
[0022] Specific implementation mode one: as figure 1 and 2 As shown, a hyperspectral super-pixel segmentation method based on principal component weighted false color synthesis and color histogram driving described in this embodiment is specifically carried out in accordance with the following steps:
[0023] Step 1: Perform principal component weighted false-color synthesis on the hyperspectral image data X of P-band M×N pixels, and convert the hyperspectral image into P-dimensional data; respectively calculate the average vector of all pixels in each dimension of the P-dimensional data ;
[0024] Step 2. Use the P-dimensional data obtained in step 1 and the average vectors of all pixels in the respective dimensions of the P-dimensional data to calculate the characteristic covariance matrix of the respective dimensions of the P-dimensional data, and calculate the characteristics of the characteristic covariance matrix of each dimension Values and eigenvectors; unitize the...
specific Embodiment approach 2
[0033]Specific implementation mode two: the difference between this implementation mode and specific implementation mode one is: the specific process of step one is:
[0034] For hyperspectral image data X=(x 1 ,x 2 ,...x i ,...,x M×N )=(X 1 ,X 2 ,...,X j ,...,X P ) T Perform principal component weighted false color synthesis, where X is a (M×N)×P dimensional matrix, x i Represents the i-th pixel in the hyperspectral image, i=1,2,...,M×N, that is, convert the M×N pixel matrix into a (M×N)×1 pixel column vector, X j Indicates the jth dimension of the image, where j=1,2,...,P;
[0035] Calculate the mean vector of all pixels in the respective dimensions of the P-dimensional data:
[0036]
[0037] Represents the mean vector of all pixels in the hyperspectral image data in each dimension.
[0038] In this embodiment, the hyperspectral image is converted into P-dimensional high-dimensional data, and by calculating the M×N groups of data relative to the mean The a...
specific Embodiment approach 3
[0039] Specific implementation mode three: the difference between this implementation mode and specific implementation mode two is: the specific process of step two is:
[0040] The feature covariance matrix of each dimension of hyperspectral image data is:
[0041]
[0042] The diagonal elements of the characteristic covariance matrix C represent the variance of each dimension; the eigenvalues and eigenvectors of the covariance matrix C of each dimension are calculated respectively, and the eigenvalues and eigenvectors of the characteristic covariance matrix C of each dimension are unitized, and the unit The transformed eigenvalue λ j and the eigenvector a j Arrange according to the order from large to small to obtain the vector λ=(λ 1 ,λ 2 ,...,λ P ) and the vector A=(a 1 ,a 2 ,...,a P ).
[0043] The off-diagonal elements of the covariance matrix measure the degree of simultaneous change between different bands. The larger the correlation coefficient, the gre...
PUM
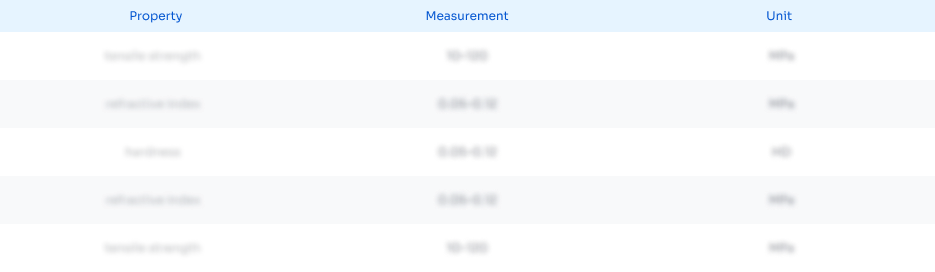
Abstract
Description
Claims
Application Information
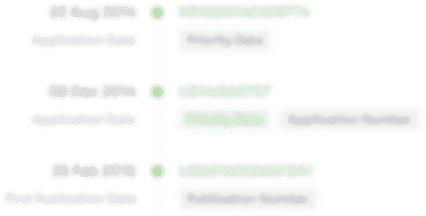
- R&D Engineer
- R&D Manager
- IP Professional
- Industry Leading Data Capabilities
- Powerful AI technology
- Patent DNA Extraction
Browse by: Latest US Patents, China's latest patents, Technical Efficacy Thesaurus, Application Domain, Technology Topic, Popular Technical Reports.
© 2024 PatSnap. All rights reserved.Legal|Privacy policy|Modern Slavery Act Transparency Statement|Sitemap|About US| Contact US: help@patsnap.com