Joint modulation recognition method based on RBM networks and BP neural network
A BP neural network and recognition method technology, applied in modulation recognition, the joint modulation recognition field based on RBM network and BP neural network, can solve the problem of slow convergence rate of neural network classification method, large impact on recognition performance, and easy clustering analysis method. Affected by noise, etc.
- Summary
- Abstract
- Description
- Claims
- Application Information
AI Technical Summary
Problems solved by technology
Method used
Image
Examples
Embodiment Construction
[0056] The present invention carries out preprocessing such as zero-mean and normalization on the modulation signal to be classified, and extracts the characteristic parameters, reduces the input dimension, and then the input layer, multi-layer hidden layer and output layer of the BP neural network according to The multi-layer RBM network is trained to obtain the initial value of the weight and bias parameters of the BP neural network, and finally the BP neural network is trained to fine-tune the parameters to identify the signal modulation mode.
[0057] The present invention will be specifically introduced below in conjunction with the accompanying drawings and specific embodiments.
[0058] refer to figure 1 , the joint modulation recognition method based on RBM network and BP neural network of the present invention The specific implementation steps are as follows:
[0059] Step1: Perform zero-mean and normalization preprocessing on the modulated signal x(n) to be classifi...
PUM
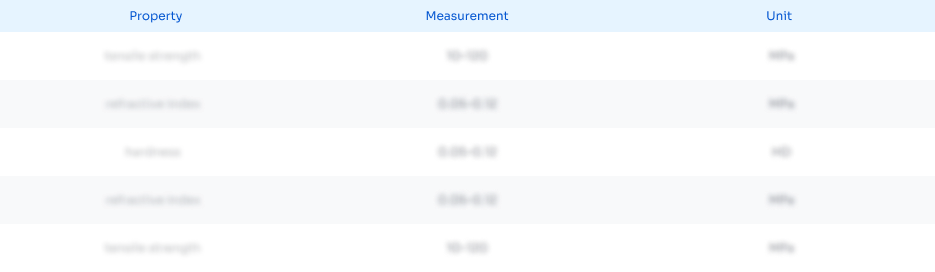
Abstract
Description
Claims
Application Information
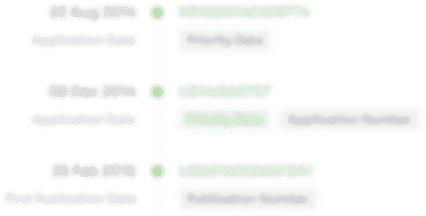
- R&D
- Intellectual Property
- Life Sciences
- Materials
- Tech Scout
- Unparalleled Data Quality
- Higher Quality Content
- 60% Fewer Hallucinations
Browse by: Latest US Patents, China's latest patents, Technical Efficacy Thesaurus, Application Domain, Technology Topic, Popular Technical Reports.
© 2025 PatSnap. All rights reserved.Legal|Privacy policy|Modern Slavery Act Transparency Statement|Sitemap|About US| Contact US: help@patsnap.com