River flow monitoring data quality control method based on chaotic neural network
A technology of flow monitoring and neural network, which is applied in the field of quality control of river flow monitoring data based on chaotic neural network, can solve the problem of not considering the time series change characteristics of flow time series data, poor quality of river flow monitoring data, and easy discovery of data, etc. question
- Summary
- Abstract
- Description
- Claims
- Application Information
AI Technical Summary
Problems solved by technology
Method used
Image
Examples
Embodiment Construction
[0041] The present invention will be further described below in conjunction with the accompanying drawings and embodiments, but not as a basis for limiting the present invention.
[0042] Example. A method for quality control of river flow monitoring data based on chaotic neural network, which consists of figure 1 shown, including the following steps:
[0043] a. Obtain the historical flow data of the regional river flow station and its upstream and downstream related stations for many years, and sort them in chronological order to obtain the time series data {x 1 ,x 2 ,x 3 ,x 4 ,x 5 ......x n};
[0044] b. Time series data {x 1 ,x 2 ,x 3 ,x 4 ,x 5 ......x n} for normalization processing to obtain normalized time series data {a 1 ,a 2 ,a 3 ,a 4 ......a n};
[0045] c. Calculate the optimal time lag τ and the optimal embedding dimension m of the normalized time series data, and use the phase space reconstruction theory to convert the one-dimensional normalize...
PUM
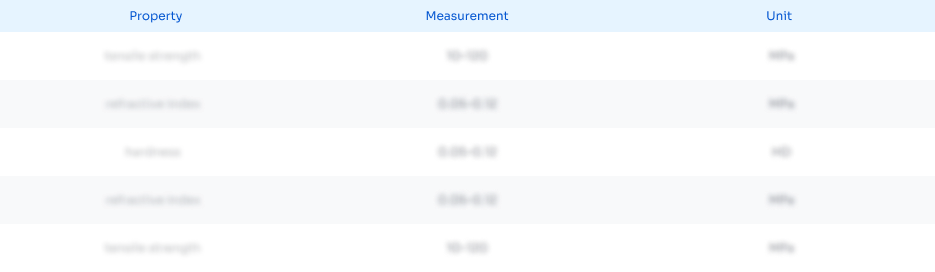
Abstract
Description
Claims
Application Information
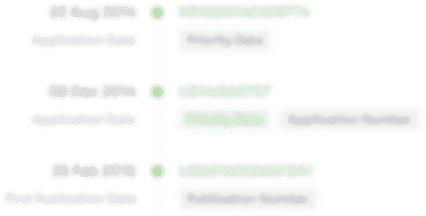
- R&D Engineer
- R&D Manager
- IP Professional
- Industry Leading Data Capabilities
- Powerful AI technology
- Patent DNA Extraction
Browse by: Latest US Patents, China's latest patents, Technical Efficacy Thesaurus, Application Domain, Technology Topic, Popular Technical Reports.
© 2024 PatSnap. All rights reserved.Legal|Privacy policy|Modern Slavery Act Transparency Statement|Sitemap|About US| Contact US: help@patsnap.com