A text implication relation recognition method based on multi-granularity information fusion
A relational recognition and multi-granularity technology, applied in neural learning methods, character and pattern recognition, text database clustering/classification, etc., can solve problems such as multi-time, lack of semantic reasoning, ignoring sentence interaction information, etc.
- Summary
- Abstract
- Description
- Claims
- Application Information
AI Technical Summary
Problems solved by technology
Method used
Image
Examples
Embodiment 1
[0081] Such as figure 1 A text implication recognition method that integrates multi-granularity information is shown, including the process of model establishment, model training and model prediction. The specific method steps are as follows:
[0082] The model building process includes: input the training sample set obtained at the input layer; for the input text pairs P and Q at the character vector layer, respectively establish a convolutional neural network (CNN) model with character granularity as the input unit, and analyze the Each word extracts character features to obtain each new word vector; in the word vector fusion layer, the Highway network layer is established, and the word vector established by the character-level convolutional neural network (CNN) model layer is passed in, and the word vector sequence based on character features is output , and then combine them with the original pre-trained word vector one by one to obtain a word vector that combines two gran...
PUM
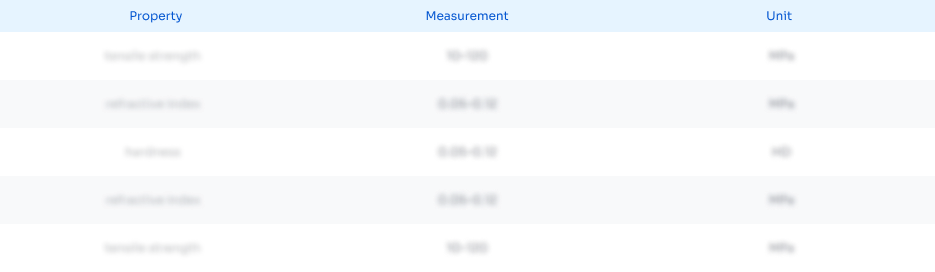
Abstract
Description
Claims
Application Information
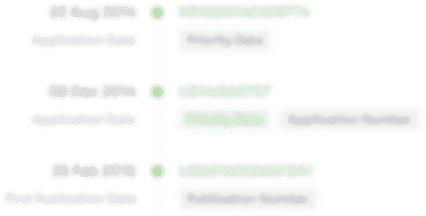
- R&D Engineer
- R&D Manager
- IP Professional
- Industry Leading Data Capabilities
- Powerful AI technology
- Patent DNA Extraction
Browse by: Latest US Patents, China's latest patents, Technical Efficacy Thesaurus, Application Domain, Technology Topic, Popular Technical Reports.
© 2024 PatSnap. All rights reserved.Legal|Privacy policy|Modern Slavery Act Transparency Statement|Sitemap|About US| Contact US: help@patsnap.com