A pneumoconiosis detection method and system based on deep learning
A technology of deep learning and detection methods, applied in image analysis, image enhancement, instruments, etc., can solve problems such as complicated method process, great energy spent by doctors, strong subjective dependence of diagnostic results, etc., to reduce medical resources and reduce waiting time Effect
- Summary
- Abstract
- Description
- Claims
- Application Information
AI Technical Summary
Problems solved by technology
Method used
Image
Examples
Embodiment 1
[0038] Such as figure 1 As shown, a deep learning-based pneumoconiosis nodule detection method provided in Embodiment 1 includes the following steps:
[0039] Step S1, the CT machine scans a complete chest image of the patient, starts the image conversion program, converts the CT image in DICOM format into a lung image in numpy array format, and reads the CT image data information.
[0040] In step 1, the CT image information refers to the patient information in the DICOM format data, the length and width of the CT image, and the interval between image pixels. Each dimension of the numpy array is n, h, w. n represents the channel of the image, h represents the height of the image, and w represents the width of the image.
[0041] Step S2, performing morphological operations to obtain a parenchymal image in which only the lung parenchyma is preserved.
[0042] In step 2, the specific operation steps of the morphological operation are as follows:
[0043] S2.1. Using a thres...
Embodiment 2
[0059] like figure 2 As shown, a deep learning-based pneumoconiosis nodule detection system provided in Embodiment 2 includes a CT machine 1, a CT image conversion module 2, a lung parenchyma segmentation module 3, a pneumoconiosis lesion area segmentation and determination module 4, and detection results The saving module (the csv file of the data saved by the detection result saving module) 5, the CT image conversion module 2, the lung parenchyma segmentation module 3, the pneumoconiosis lesion area segmentation and judgment module are programmed in Python language; the CT image scanned by the CT machine is input into the CT image The conversion module 2, the input of the lung parenchyma segmentation module 3 is the output of the CT image conversion module 2, the input of the pneumoconiosis lesion area segmentation and judgment module 4 is the output of the lung parenchyma segmentation module 3, and the detection result preservation module 5 is saved in the database The dat...
PUM
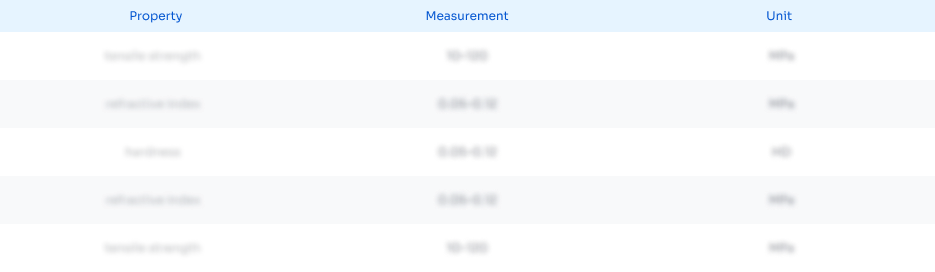
Abstract
Description
Claims
Application Information
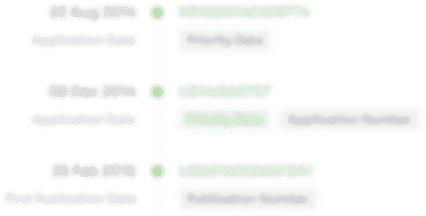
- R&D
- Intellectual Property
- Life Sciences
- Materials
- Tech Scout
- Unparalleled Data Quality
- Higher Quality Content
- 60% Fewer Hallucinations
Browse by: Latest US Patents, China's latest patents, Technical Efficacy Thesaurus, Application Domain, Technology Topic, Popular Technical Reports.
© 2025 PatSnap. All rights reserved.Legal|Privacy policy|Modern Slavery Act Transparency Statement|Sitemap|About US| Contact US: help@patsnap.com