An Adversarial Robust Image Feature Extraction Method Based on Variational Spherical Projection
A technology of image feature extraction and spherical projection, applied in the field of image processing, can solve problems such as the decline of model expression ability, system error authorization of lawbreakers, and life security threats.
- Summary
- Abstract
- Description
- Claims
- Application Information
AI Technical Summary
Problems solved by technology
Method used
Image
Examples
Embodiment Construction
[0078] The present invention will be further described below in conjunction with specific examples.
[0079] Such as figure 1 As shown, the anti-robust image feature extraction method based on variational spherical projection provided in this embodiment includes the following steps:
[0080] 7) Repeat the process from step 2) to step 6) until convergence to obtain a deep feature extraction model; when applying, use the mean parameter of the parameter encoding process as a feature to obtain highly distinguishable features.
[0081] 1) Model initialization, including the following steps:
[0082] Define the model structure f(·|W of the deep feature extractor f ,b f ), and an unbiased linear classifier g(·|W g ), where the deep feature extractor has L layers corresponding to L weight matrices and bias term where W f l Represents the weight matrix of layer l, W f L Represents the weight matrix of the last layer, Indicates the l-th layer bias term, Represents the la...
PUM
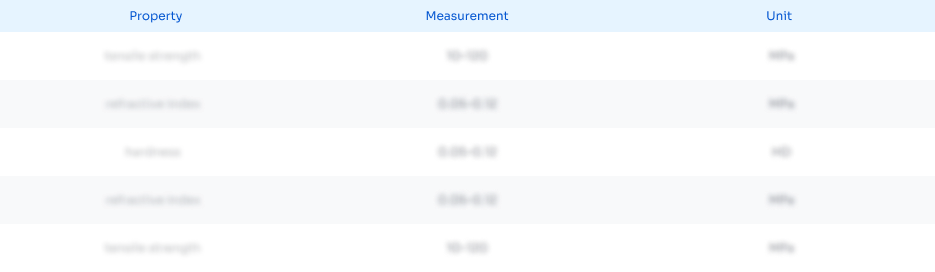
Abstract
Description
Claims
Application Information
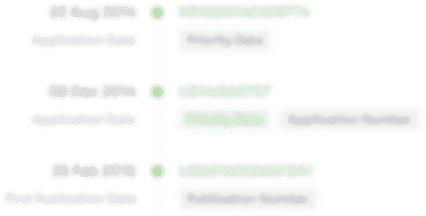
- R&D Engineer
- R&D Manager
- IP Professional
- Industry Leading Data Capabilities
- Powerful AI technology
- Patent DNA Extraction
Browse by: Latest US Patents, China's latest patents, Technical Efficacy Thesaurus, Application Domain, Technology Topic, Popular Technical Reports.
© 2024 PatSnap. All rights reserved.Legal|Privacy policy|Modern Slavery Act Transparency Statement|Sitemap|About US| Contact US: help@patsnap.com