Time series classification method and device based on characteristic sampling
A time series and classification method technology, applied in the field of data processing, can solve the problems of algorithm generalization and accuracy that cannot meet the time series classification problem, cannot deal with local similarity problems, and the performance of a single model is weak, etc., to achieve Good effect, high classification accuracy and strong generalization
- Summary
- Abstract
- Description
- Claims
- Application Information
AI Technical Summary
Problems solved by technology
Method used
Image
Examples
Embodiment 1
[0052] like figure 1 As shown, the time series classification method based on feature sampling provided by the embodiment of the present invention may include the following steps:
[0053] Step S101: converting the time series data set used for training into a training data set with equal-length features by using a feature sampling method, and converting the time series data set used for testing into a test data set with equal-length features;
[0054] Step S102: using the ensemble learning classification method, using the training data set with equal-length features to perform model training;
[0055] Step S103: Use the trained model to perform time series classification on the test data set with features of equal length.
[0056] The present invention mainly aims at the feature selection problem of the time series problem. Given a sample set of time series, each sample contains a time series X i ={x(t)|t∈{1,2,,T}} and the classification label corresponding to the time ser...
Embodiment 2
[0060] On the basis of the time series classification method based on feature sampling provided in Embodiment 1, the feature sampling method in step S101 is a segmented feature sampling method, which can be specifically implemented in the following ways:
[0061] First, set the segment length l 1 , the number of segments m 1 and interval between segments g 1 ;
[0062] Subsequently, for each piece of time series data in the time series dataset, such as Figure 2a As shown, sampling is performed from the start position of the time series data, and continuous l is selected 1 time series data as the first piece of feature data, and the starting position of each piece of sampling after that is the starting position of the previous piece of sampling plus the interval between segments g 1 , select l for each segment 1 sequence data, after sampling, convert each piece of time series data into m 1 feature data (that is, sub-time series T1, T2, T3...). Preferably, l 1 =5,g 1 =...
Embodiment 3
[0064] On the basis of the time series classification method based on feature sampling provided in Embodiment 2, the ensemble learning classification method adopted in step S102 is a random forest classifier based on bagging.
[0065] After the sampling method is determined, the selection and design of the classifier is also an important research content of the present invention. The current mainstream classification methods include basic classification methods such as decision trees and logistic regression, as well as integrated learning methods such as XGboost and gradient boosting trees. The realization of algorithms such as decision tree and logistic regression is easy to write, but the effect is worse than the integrated learning classification method on the accuracy rate, so in the selection of the classifier, the present invention adopts the integrated learning classification method, combined with the experimental results, the random forest classifier Works best. The m...
PUM
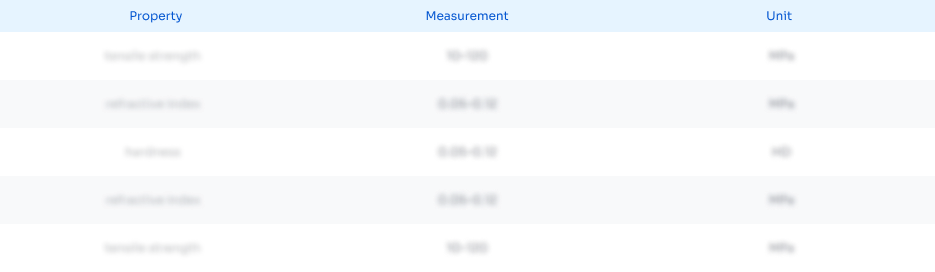
Abstract
Description
Claims
Application Information
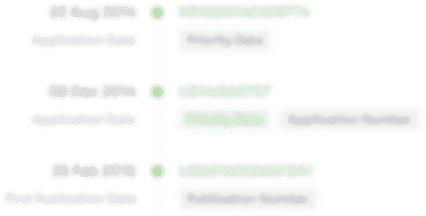
- R&D Engineer
- R&D Manager
- IP Professional
- Industry Leading Data Capabilities
- Powerful AI technology
- Patent DNA Extraction
Browse by: Latest US Patents, China's latest patents, Technical Efficacy Thesaurus, Application Domain, Technology Topic, Popular Technical Reports.
© 2024 PatSnap. All rights reserved.Legal|Privacy policy|Modern Slavery Act Transparency Statement|Sitemap|About US| Contact US: help@patsnap.com