End-to-end game robot generation method and system based on multi-category imitation learning
A multi-category, robot technology, applied in manipulators, program-controlled manipulators, manufacturing tools, etc., can solve problems such as poor generalization ability, insufficient scientific level division of game robots, and inability of robot games to meet high-quality interactive games.
- Summary
- Abstract
- Description
- Claims
- Application Information
AI Technical Summary
Problems solved by technology
Method used
Image
Examples
Embodiment 1
[0041] This embodiment is based on the end-to-end game robot generation method of multi-category imitation learning, including:
[0042] A player sample database is set up, the player sample database includes: player status characteristics of players of various skill levels during game play, game actions performed by players, and several predefined skill level tags;
[0043] The policy generator, the policy discriminator, and the policy classifier form an adversarial network. The policy generator, the policy discriminator, and the policy classifier are all multi-layer neural networks. The policy generator performs imitation learning in the adversarial network, and the policy generation The machine obtains the game strategy similar to the game behavior of players of different skill levels, and then generates a game robot;
[0044] The policy generator input consists of generator state features S g , any technical grade label C i The generated state label pair (S g ,C i ), t...
Embodiment 2
[0067] This embodiment is based on the end-to-end game robot generation method based on multi-category imitation learning. On the basis of Embodiment 1, an effective convolutional neural network is obtained based on transfer learning training, and the effective convolutional neural network is used to obtain the player game image from each frame. 1. Extract effective features from the generated game images of each frame to obtain the player state features corresponding to the player game images in each frame and the generator state features corresponding to the generated game images in each frame.
[0068] In this embodiment, the effective convolutional neural network processes the original high-dimensional game image data, extracts more effective features from it as training data for imitation learning, and then obtains a game robot with a higher degree of imitation of the player's game behavior.
Embodiment 3
[0070] This embodiment is based on an end-to-end game robot generation method based on multi-category imitation learning. On the basis of the above-mentioned embodiment 1 or 2, the policy discriminator D ω and policy classifier C ψ The gradient update of the ADAM can use the momentum gradient of ADAM or the update method of the general gradient. And the policy generator can be G θ Stable incremental policy gradient update methods such as PPO or TRPO in reinforcement learning can be used, and techniques such as GAE can be used to weaken the influence of variance on gradient updates. This end-to-end multi-category imitation learning based on the auxiliary classification generation confrontation network mechanism, after continuous training, the policy generator G θ It can be a multi-category strategy approximator, generating game strategies similar to the player's game behavior under multiple categories.
[0071] In this embodiment, when the policy discriminator D is close to ...
PUM
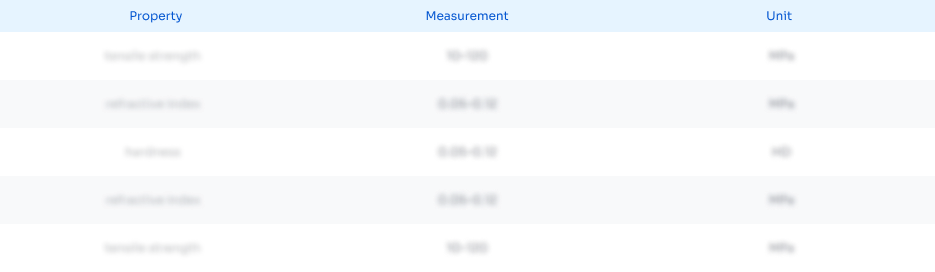
Abstract
Description
Claims
Application Information
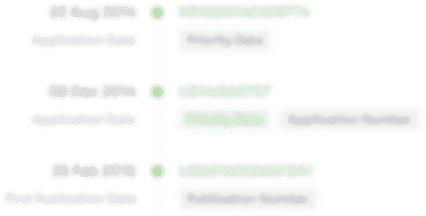
- R&D Engineer
- R&D Manager
- IP Professional
- Industry Leading Data Capabilities
- Powerful AI technology
- Patent DNA Extraction
Browse by: Latest US Patents, China's latest patents, Technical Efficacy Thesaurus, Application Domain, Technology Topic, Popular Technical Reports.
© 2024 PatSnap. All rights reserved.Legal|Privacy policy|Modern Slavery Act Transparency Statement|Sitemap|About US| Contact US: help@patsnap.com