Video event recognition method based on deep residual long-short term memory network
A long-short-term memory and video event technology, applied in character and pattern recognition, biological neural network models, computer components, etc., can solve problems such as small distance between event classes, gradient disappearance, camera viewing angle changes, etc., to achieve good generalization The effects of ability and discrimination, shortening the intra-class distance, and improving discrimination
- Summary
- Abstract
- Description
- Claims
- Application Information
AI Technical Summary
Problems solved by technology
Method used
Image
Examples
specific Embodiment approach
[0025] Shown in conjunction with accompanying drawing is the embodiment of a kind of video event recognition method based on depth residual long short-term memory network of the present invention, comprises the following steps:
[0026] Step 1) Design of spatio-temporal feature data connection unit: the spatio-temporal feature data is synchronously analyzed by LSTM to form a spatio-temporal feature data link unit DLSTM;
[0027] Such as figure 1 As shown, the specific steps include:
[0028] (1) Receive data: First, two LSTM units are used, which are respectively denoted as SLSTM and TLSTM, and SLSTM receives the feature h from the spatial CNN network SL , the TLSTM receives the feature h from the temporal CNN network TL ;
[0029] (2) Activation function conversion: Before receiving input, the LSTM unit needs to use a nonlinear activation function to process the input data, using the ReLU activation function, SLSTM and TLSTM are transformed by the ReLU activation function ...
PUM
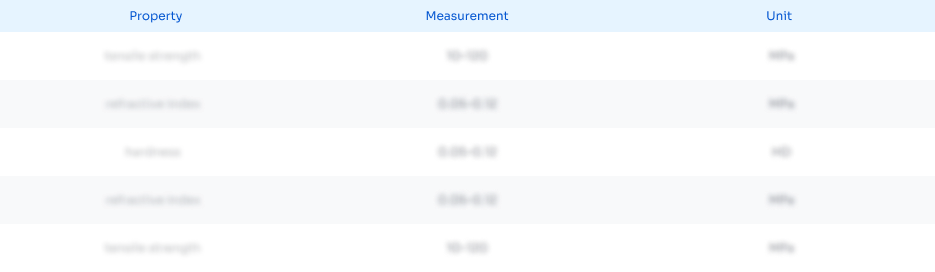
Abstract
Description
Claims
Application Information
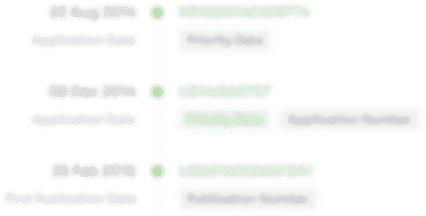
- R&D
- Intellectual Property
- Life Sciences
- Materials
- Tech Scout
- Unparalleled Data Quality
- Higher Quality Content
- 60% Fewer Hallucinations
Browse by: Latest US Patents, China's latest patents, Technical Efficacy Thesaurus, Application Domain, Technology Topic, Popular Technical Reports.
© 2025 PatSnap. All rights reserved.Legal|Privacy policy|Modern Slavery Act Transparency Statement|Sitemap|About US| Contact US: help@patsnap.com