Structural health monitoring abnormal data diagnosis method based on computer vision and deep learning technology
A computer vision and deep learning technology, applied in the fields of civil engineering structural health monitoring, machine learning, and signal processing, can solve the problems of inability to meet the accuracy and efficiency requirements of online early warning and structural state assessment, high cost, and low degree of automation. Improve efficiency and reliability, reduce manual participation, and facilitate the process
- Summary
- Abstract
- Description
- Claims
- Application Information
AI Technical Summary
Problems solved by technology
Method used
Image
Examples
specific Embodiment approach 1
[0023] Specific implementation mode 1: The abnormal data diagnosis method of structural health monitoring based on computer vision and deep learning technology in this implementation mode, such as Figure 7 shown, including:
[0024] Step 1. Convert the monitoring data used for training from time series data to time domain response image data and frequency domain response image data through data visualization processing; according to the time domain response image data and frequency domain response image data corresponding to the same data segment Form a dual-channel time-frequency response diagram; select samples from the dual-channel time-frequency response diagram and mark the abnormal type of the sample to form a training set; data visualization is the process of turning data into visible curves and charts, such as in Figure 1(a) It is to convert time domain and frequency domain data into time domain and frequency domain images.
[0025] Step 2. Input the training set int...
specific Embodiment approach 2
[0031] Specific implementation mode two: the difference between this implementation mode and specific implementation mode one is that step one is specifically:
[0032] Step 11. Cut the monitoring data to be diagnosed into n data segments d according to the time interval w, and generate a data set D{d};
[0033] Step 12, draw the time-domain corresponding diagram and frequency-domain response diagram of each data segment d in the data set D respectively, and generate a dual-channel time-frequency response diagram p according to the time-domain corresponding diagram and frequency-domain response diagram of the same data segment, Form a data set D{d,p};
[0034] Step 13: Randomly select m picture samples p from D to form a training set S{p};
[0035] Step 14. In the training set S, evaluate the abnormal type of the sample p according to the time and frequency domain response characteristics, and label p with a label L;
[0036]Step 15. Repeat step 14 until all m samples in the...
specific Embodiment approach 3
[0039] Specific embodiment three: the difference between this embodiment and specific embodiment one or two is that in the time-frequency response graph p, the R channel is used to represent the time domain response graph, and the G channel is used to represent the frequency domain response graph, and the time domain response graph and the frequency domain Areas where the response plots overlap are set to black.
[0040] The parameters are the same as those in Embodiment 1 or 2.
PUM
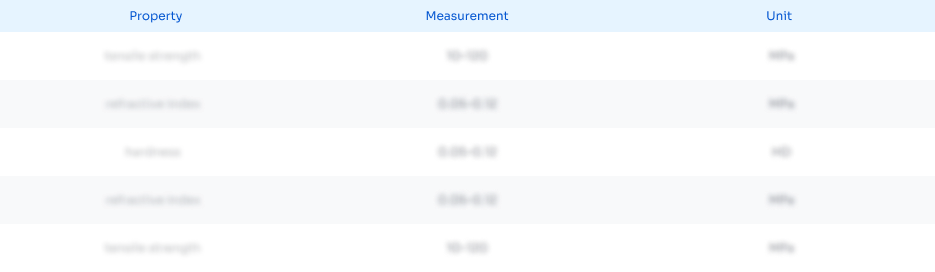
Abstract
Description
Claims
Application Information
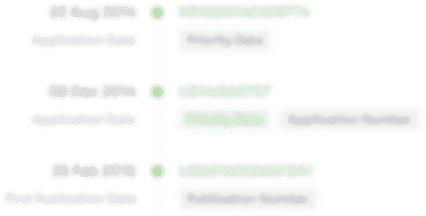
- R&D Engineer
- R&D Manager
- IP Professional
- Industry Leading Data Capabilities
- Powerful AI technology
- Patent DNA Extraction
Browse by: Latest US Patents, China's latest patents, Technical Efficacy Thesaurus, Application Domain, Technology Topic, Popular Technical Reports.
© 2024 PatSnap. All rights reserved.Legal|Privacy policy|Modern Slavery Act Transparency Statement|Sitemap|About US| Contact US: help@patsnap.com