Target detection method based on Faster-RCNN reinforcement learning
A technology of the image to be tested and the basic network, which is applied in the field of image processing, can solve problems such as lighting, occlusion, background confusion and scale problems, and achieve the effect of increasing the number of candidate regions, improving detection accuracy, and improving performance
- Summary
- Abstract
- Description
- Claims
- Application Information
AI Technical Summary
Problems solved by technology
Method used
Image
Examples
Embodiment Construction
[0034] The specific embodiments of the present invention will be further described below in conjunction with the accompanying drawings.
[0035] The present application discloses a target detection method based on Faster-RCNN reinforcement learning, where the size of the target to be detected is different, for example, the target is a pedestrian, a vehicle, a flame, and the like. Faster-RCNN (FasterRegion-based Convolutional Neural Network, faster region-based convolutional neural network) in the present invention includes convolutional neural network, RPN (Region Proposal Networks, candidate area network) and classifier, convolutional neural network Can be a residual network, the convolutional neural network includes M network layers, M is a positive integer and M≥2, the basic model of the convolutional neural network used in the present invention is ResNet-50, then M=50, the classification used The classifier is a softmax classifier.
[0036] The method disclosed in the pre...
PUM
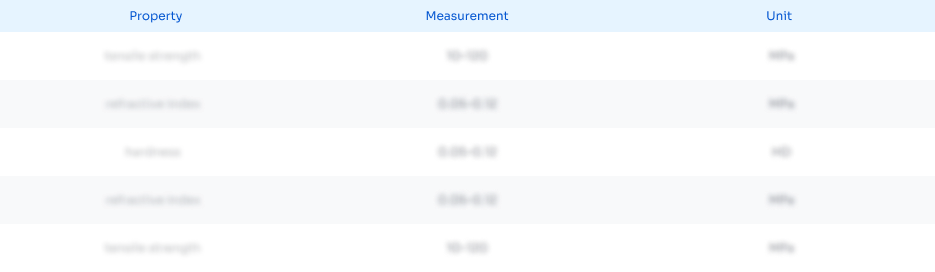
Abstract
Description
Claims
Application Information
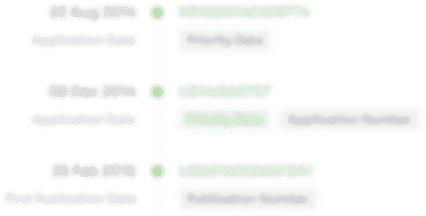
- R&D Engineer
- R&D Manager
- IP Professional
- Industry Leading Data Capabilities
- Powerful AI technology
- Patent DNA Extraction
Browse by: Latest US Patents, China's latest patents, Technical Efficacy Thesaurus, Application Domain, Technology Topic, Popular Technical Reports.
© 2024 PatSnap. All rights reserved.Legal|Privacy policy|Modern Slavery Act Transparency Statement|Sitemap|About US| Contact US: help@patsnap.com