Image denoising method based on expansion convolution
An image and convolution technology, which is applied in the field of image denoising, can solve the problems of too deep layers, long time required, and reduced efficiency, so as to reduce time and improve efficiency.
- Summary
- Abstract
- Description
- Claims
- Application Information
AI Technical Summary
Problems solved by technology
Method used
Image
Examples
Embodiment Construction
[0032] In order to further illustrate the technical means and effects adopted by the present invention to achieve the intended purpose, the specific implementation, structural features and effects of the present invention will be described in detail below in conjunction with the accompanying drawings and examples.
[0033] In the existing technology, there is not only the problem of deep network layers, but also the "grid problem". Since the expansion convolution fills zeros between two pixels in the convolution kernel, the feeling of the convolution kernel is The field only covers regions with a checkerboard pattern - only sampling locations with non-zero values, so some neighborhood information is lost. The "grid problem" gets worse when the dilation factor increases, usually at higher layers with larger receptive fields: the kernels are too sparse to cover any local information because the non-zero values are too far away.
[0034] In order to solve the deep network layer...
PUM
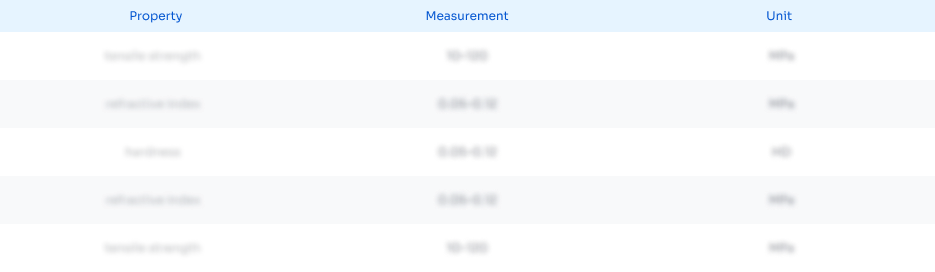
Abstract
Description
Claims
Application Information
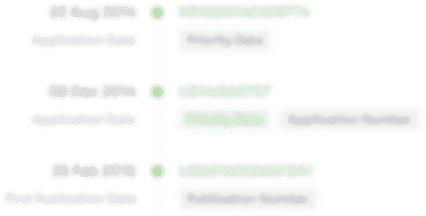
- R&D Engineer
- R&D Manager
- IP Professional
- Industry Leading Data Capabilities
- Powerful AI technology
- Patent DNA Extraction
Browse by: Latest US Patents, China's latest patents, Technical Efficacy Thesaurus, Application Domain, Technology Topic, Popular Technical Reports.
© 2024 PatSnap. All rights reserved.Legal|Privacy policy|Modern Slavery Act Transparency Statement|Sitemap|About US| Contact US: help@patsnap.com