Improved Lasso+RBF neural network combined prediction model
A neural network and combined forecasting technology, applied in biological neural network models, forecasting, neural architecture, etc., can solve problems such as inaccurate analysis and performance bottlenecks, and achieve the effect of improving accuracy and reducing imbalance
- Summary
- Abstract
- Description
- Claims
- Application Information
AI Technical Summary
Problems solved by technology
Method used
Image
Examples
Embodiment Construction
[0058] The preferred embodiments of the present invention will be described in detail below with reference to the accompanying drawings.
[0059] The improved Lasso+RBF neural network combined prediction model of the present invention, the prediction process is:
[0060] First, analyze the introduction of telecom customer information and telecom customer life cycle, and give the definition of each life cycle stage. According to this definition, telecom customers are divided into acquisition stage, promotion stage, mature stage, decline stage and loss stage. To define, customers in the loss stage are divided into the acquisition stage, promotion stage, maturity stage and decline stage; secondly, for the sub-stages of the loss stage, the Lasso regression algorithm is used to extract features and obtain the characteristic equation, and the extracted non-zero coefficients The corresponding feature is used as the input of the RBF neural network, and then the center point of the dat...
PUM
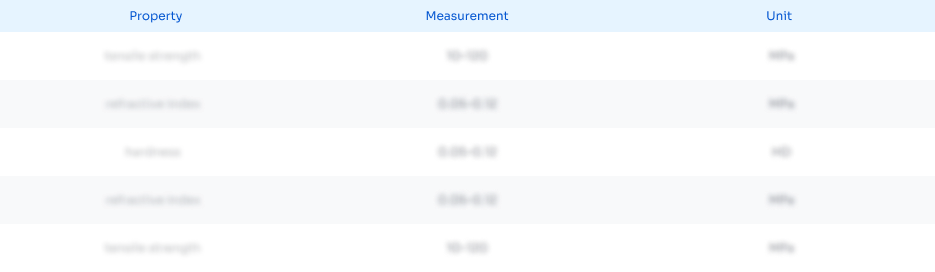
Abstract
Description
Claims
Application Information
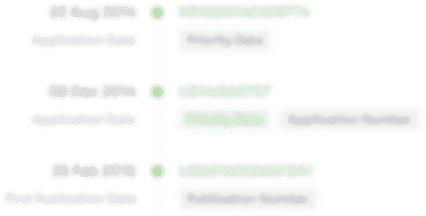
- R&D Engineer
- R&D Manager
- IP Professional
- Industry Leading Data Capabilities
- Powerful AI technology
- Patent DNA Extraction
Browse by: Latest US Patents, China's latest patents, Technical Efficacy Thesaurus, Application Domain, Technology Topic.
© 2024 PatSnap. All rights reserved.Legal|Privacy policy|Modern Slavery Act Transparency Statement|Sitemap