MIL-based medical image lesion point accurate marking method
A medical image and lesion technology, applied in the field of medical image processing, can solve the problems of scarce samples, precise marking of lesion areas, and small number, and achieve the effects of reducing workload, high universality, and high efficiency
- Summary
- Abstract
- Description
- Claims
- Application Information
AI Technical Summary
Problems solved by technology
Method used
Image
Examples
Embodiment Construction
[0023] The present invention will be further described below in conjunction with the accompanying drawings and specific embodiments.
[0024] A method for accurately labeling medical image focus points based on MIL, comprising the following steps:
[0025] Step 1: Collect medical image data sets, which are divided into positive sample sets and negative sample sets;
[0026] Collect several images of different types of lesion points through the camera of medical equipment, and select images with clear pictures at a certain interval of images as the original data set; select a certain number of pictures, and make corresponding image labels for subsequent training.
[0027] Step 2: Initialize the classification model;
[0028] Select samples with labels for supervised learning, and initialize the classification model; the classification model chooses a model based on the LeNet-based accurate classification of medical image lesions. The classification model is not limited to LeNe...
PUM
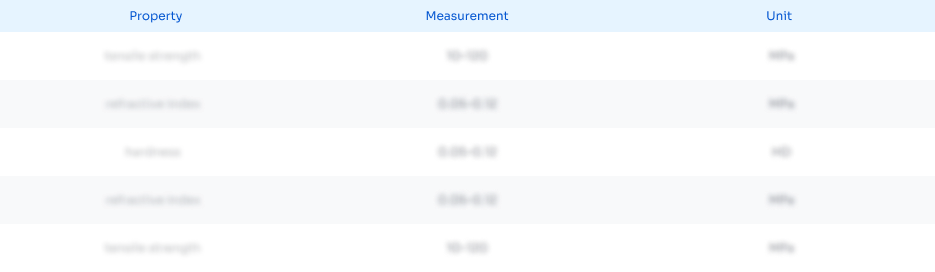
Abstract
Description
Claims
Application Information
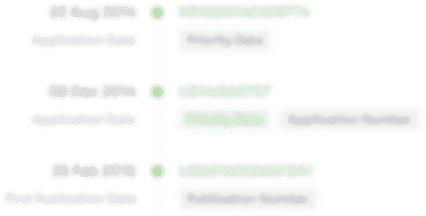
- R&D Engineer
- R&D Manager
- IP Professional
- Industry Leading Data Capabilities
- Powerful AI technology
- Patent DNA Extraction
Browse by: Latest US Patents, China's latest patents, Technical Efficacy Thesaurus, Application Domain, Technology Topic.
© 2024 PatSnap. All rights reserved.Legal|Privacy policy|Modern Slavery Act Transparency Statement|Sitemap