An end-to-end based human body articulation point detection and classification method
A technology of human joints and classification methods, applied in neural learning methods, instruments, biological neural network models, etc., can solve problems such as weak robustness and unintuitive display of results, and achieve enhanced correlation and spatial position correlation , the effect of high precision estimation effect
- Summary
- Abstract
- Description
- Claims
- Application Information
AI Technical Summary
Problems solved by technology
Method used
Image
Examples
Embodiment 1
[0034] The end-to-end human joint point detection and classification method includes the following steps in sequence:
[0035] S1. Construct a deep learning network based on stacked hourglass and conditional random field theory, and initialize the network;
[0036] S2. Train the network parameters, and obtain network weight parameters suitable for human body pose estimation through forward propagation;
[0037] S3. Input the preprocessed image into the trained hourglass neural network, obtain the heat map distribution of the corresponding joint points through forward propagation, and then use the conditional random field model to strengthen the spatial position relationship between each node, and finally Obtain the probability and statistics distribution of the appearance of each node, and connect the joint points with the output prediction results using preset rules, so as to realize end-to-end human pose estimation.
[0038] Considering adapting to joint point regression in...
Embodiment 2
[0060] The present invention uses 25,000 pictures in the MPII Human Pose Dataset as training and testing samples, and uses the center position of each picture as the estimated position of the body. And the preprocessing work of data augmentation such as simple scale scaling, rotation, adding noise, etc. is performed on the training pictures.
[0061] figure 1 It shows the basic flow chart of the deep learning network training and testing process based on stacked hourglass and conditional random field theory proposed by the present invention. Such as figure 1 As shown, in the training phase of the network, the image must first be preprocessed, and each video frame or image is cut into a size of 256x256 and then input into the network. For the training phase, first initialize the network parameters, set the initial learning rate to 0.0007, and adjust the number of human body nodes according to the needs, which is set to 16 here. In the forward propagation process, the paramet...
PUM
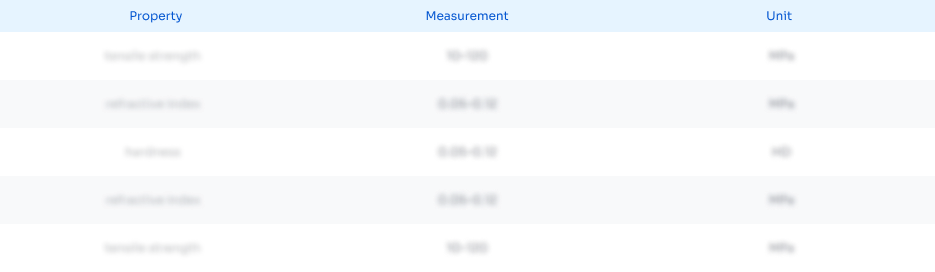
Abstract
Description
Claims
Application Information
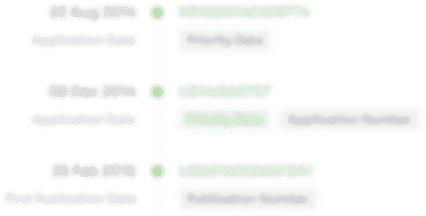
- R&D Engineer
- R&D Manager
- IP Professional
- Industry Leading Data Capabilities
- Powerful AI technology
- Patent DNA Extraction
Browse by: Latest US Patents, China's latest patents, Technical Efficacy Thesaurus, Application Domain, Technology Topic, Popular Technical Reports.
© 2024 PatSnap. All rights reserved.Legal|Privacy policy|Modern Slavery Act Transparency Statement|Sitemap|About US| Contact US: help@patsnap.com