Auxiliary diagnosis method and system based on deep learning of electroencephalograms
A deep learning and auxiliary diagnosis technology, applied in the field of disease diagnosis, can solve the problems of low diagnosis efficiency and low accuracy of automatic diagnosis of epilepsy, and achieve the effect of improving diagnosis efficiency and ensuring accuracy
- Summary
- Abstract
- Description
- Claims
- Application Information
AI Technical Summary
Problems solved by technology
Method used
Image
Examples
Embodiment 1
[0052] refer to figure 1 , the present embodiment provides a method for auxiliary diagnosis based on EEG deep learning, including:
[0053] S10: Obtain the collected EEG sample data, integrate the EEG sample data into a preset normalized model, and obtain normalized EEG integer data;
[0054] S20: Convert the normalized EEG integer data into a word embedding vector according to a preset word embedding model;
[0055]S30: Perform feature extraction on the word embedding vector according to a preset deep learning model, and perform time stamping and identification and diagnosis on the extracted features;
[0056] S40: According to the time stamp and identification diagnosis, output the probability of disease onset, and distinguish the EEG sample data whose disease onset probability exceeds a preset probability.
[0057] In this application, the corresponding medical equipment is used to diagnose the diseased person, that is, the corresponding diagnostic steps and systems are s...
Embodiment 2
[0092] refer to figure 2 , the present embodiment provides an auxiliary diagnosis system based on EEG deep learning, including:
[0093] Input normalization module 21: used to obtain the collected EEG sample data, integrate the EEG sample data into a preset normalization model, and obtain normalized EEG integer data;
[0094] Word embedding module 22: for converting the normalized EEG integer data into a word embedding vector according to a preset word embedding model;
[0095] Deep learning module 23: used to perform feature extraction on the word embedding vector according to a preset deep learning model, and perform time stamping and identification and diagnosis on the extracted features;
[0096] Output module 24: used for outputting the disease onset probability according to the time stamp and identification diagnosis, and distinguishing the EEG sample data whose disease onset probability exceeds a preset probability.
[0097] In this embodiment, the word embedding mod...
Embodiment 3
[0113] refer to image 3 , this embodiment provides a flow chart of an auxiliary diagnosis method based on deep learning of EEG. The patient is scanned by a scanner to obtain an EEG sample image, and the format and value of the scanner input data are integrated into the standardized In the model, the input matrix format is obtained; through the word embedding model, the EEG database data is converted and compressed into a low-latitude word embedding vector with features; and the word embedding vector is marked and identified and diagnosed through the deep learning model; finally, the The output module gives the seizure flag of 0 or 1, and the probability of the disease, wherein, when it is 0, it means no epilepsy, and 1 means epileptic seizure.
[0114] Taking this example as an example, the training set included 118 patients with seizure EEG and 146 patients with normal background EEG. The total recording time was 330.08 hours. The test set included 38 patients with seizure E...
PUM
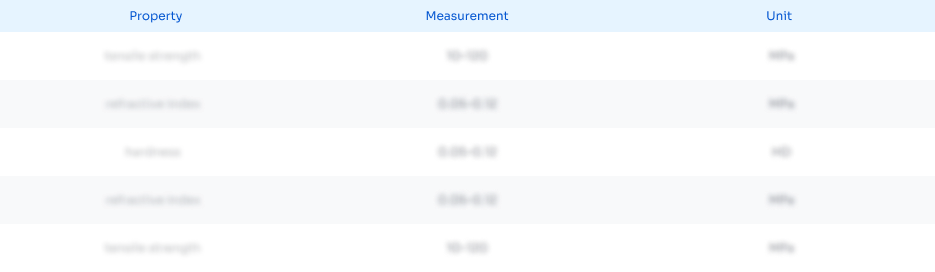
Abstract
Description
Claims
Application Information
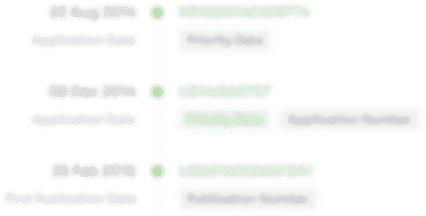
- R&D Engineer
- R&D Manager
- IP Professional
- Industry Leading Data Capabilities
- Powerful AI technology
- Patent DNA Extraction
Browse by: Latest US Patents, China's latest patents, Technical Efficacy Thesaurus, Application Domain, Technology Topic, Popular Technical Reports.
© 2024 PatSnap. All rights reserved.Legal|Privacy policy|Modern Slavery Act Transparency Statement|Sitemap|About US| Contact US: help@patsnap.com