Short-term bus passenger flow forecasting method based on in-depth learning and passenger behavior model
A technology of deep learning and forecasting methods, applied in forecasting, complex mathematical operations, instruments, etc., can solve the problems of forecasting, errors, and interaction mechanisms that have not been fully exploited and utilized, and achieve the effect of improving forecasting accuracy and accuracy.
- Summary
- Abstract
- Description
- Claims
- Application Information
AI Technical Summary
Problems solved by technology
Method used
Image
Examples
Embodiment
[0055] The present embodiment provides a short-term bus passenger flow prediction method based on deep learning and passenger behavior patterns, and the method includes the following parts:
[0056] 1 Identification and Feature Extraction of Influencing Factors of Bus Passenger Flow
[0057] In the model of bus passenger flow prediction, the present invention increases and designs bus card swiping type, passenger's The three internal influencing factors related to the internal components of bus passenger flow are bus line dependence and passenger travel behavior, in order to further subdivide the total passenger flow through the interaction of internal and external factors, and establish a deep learning algorithm to extract various internal components of passenger flow The characteristics of the components can improve the accuracy of bus passenger flow forecasting.
[0058] 1.1 External Factors Affecting Bus Passenger Flow
[0059] Combined with the statistical data of bus I...
PUM
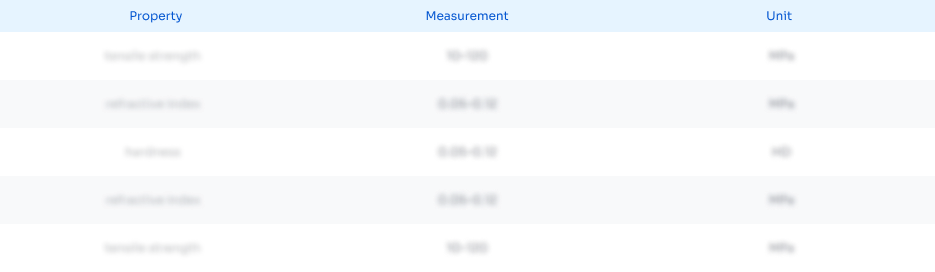
Abstract
Description
Claims
Application Information
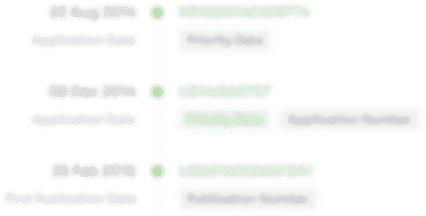
- Generate Ideas
- Intellectual Property
- Life Sciences
- Materials
- Tech Scout
- Unparalleled Data Quality
- Higher Quality Content
- 60% Fewer Hallucinations
Browse by: Latest US Patents, China's latest patents, Technical Efficacy Thesaurus, Application Domain, Technology Topic, Popular Technical Reports.
© 2025 PatSnap. All rights reserved.Legal|Privacy policy|Modern Slavery Act Transparency Statement|Sitemap|About US| Contact US: help@patsnap.com