Motion planning method based on driving habit learning
A technology of motion planning and driving habits, applied in measuring devices, instruments, surveying and navigation, etc., can solve problems such as difficulty in describing road structure and traffic rules, mismatching, mismatching, etc., and achieve complex road and traffic conditions , Reduce data redundancy, reduce the effect of data transmission volume
- Summary
- Abstract
- Description
- Claims
- Application Information
AI Technical Summary
Problems solved by technology
Method used
Image
Examples
Embodiment 1
[0106] (1) Specific steps:
[0107] Since the collected artificial driving trajectory may be very long, the trajectory is segmented first, such as Figure 11 shown.
[0108] Perform a least-squares fit on each trajectory:
[0109] y=a 0 +a 1 x+a 2 x 2 +...+a m x m
[0110]
[0111] And find the median error of each trajectory:
[0112]
[0113]
[0114] The trajectory point least squares fitting is as follows Figure 12 shown.
[0115] In the same way, the center error of the head pointing, curvature, speed and other information of each trajectory can be calculated. Then, the weight value of each point on the trajectory σ xy Indicates the median error of the track point of the driving track A; σ θ Indicates the medium error of the head pointing of the driving trajectory A; σ k Indicates the median error of the curvature of the driving trajectory A; σ v Indicates the medium error of the speed of the driving trajectory A; ∝, β, γ, is a constant.
[01...
Embodiment 2
[0127] We can also obtain intersection and other information by processing the trajectory image. Such as Figure 16 As shown, the area with the highest trajectory density thermal value is the intersection area, and we can extract the intersection area through the image operation of expansion and edge detection, as shown in the red box in the figure.
[0128] Specific steps: take the boundary line as a cross section, and extract preview points of key positions such as intersections. The subsequent process is consistent with that in Example 1.
[0129] 3. Summary of key technical points of the present invention
[0130] (1) The method and process of collecting manual driving trajectories by crowdsourcing, assigning different weight values to trajectories from different sources, and projecting the trajectory points onto the pixel plane, and obtaining the position distribution of the preview points through graphic processing.
[0131] (2) The definition of the preview point i...
PUM
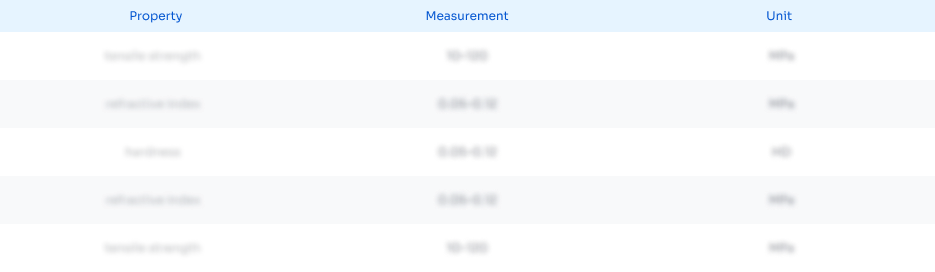
Abstract
Description
Claims
Application Information
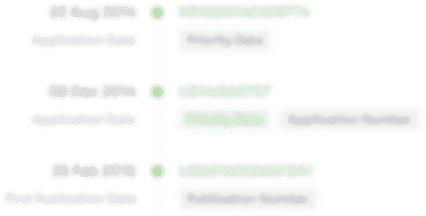
- R&D Engineer
- R&D Manager
- IP Professional
- Industry Leading Data Capabilities
- Powerful AI technology
- Patent DNA Extraction
Browse by: Latest US Patents, China's latest patents, Technical Efficacy Thesaurus, Application Domain, Technology Topic, Popular Technical Reports.
© 2024 PatSnap. All rights reserved.Legal|Privacy policy|Modern Slavery Act Transparency Statement|Sitemap|About US| Contact US: help@patsnap.com