Man-machine multi-round dialogue method oriented to travel field
A human-machine and domain technology, applied in the field of human-machine dialogue, can solve the problem of difficulty in extracting intention information and slot information, and achieve the effect of overcoming limitations
- Summary
- Abstract
- Description
- Claims
- Application Information
AI Technical Summary
Problems solved by technology
Method used
Image
Examples
specific Embodiment approach 1
[0021] Specific implementation mode one: combine figure 1 This embodiment will be described. A human-machine multi-round dialogue method oriented to the field of travel described in this embodiment, the specific steps of the method are:
[0022] Step 1. Standardize the user's current question, and then, if there are clear demonstrative pronouns or lack of sentence substructure in the current question, according to the slot information involved in the previous interaction with the user, the instructions in the current question Pronouns and missing sentence substructures are replaced or filled in; current questions are processed;
[0023] Step 2. Use DAN, CNN or BLSTM model to obtain the intention information of the current question after step 1 processing: input the current question after step 1 processing into DAN, CNN or BLSTM model; the output of DAN, CNN or BLSTM model is passed through softmax The operation obtains the intention probability of the current question, and u...
specific Embodiment approach 2
[0027] Specific implementation mode two: combination figure 2 This embodiment will be described. This embodiment further defines the human-machine multi-round dialogue method for the travel field described in the first embodiment; the specific process of using the DAN model to obtain the intention information of the current question after step one is as follows:
[0028] Before using deep learning to conduct experiments, the present invention first uses the word2vec open-sourced by Google to train word vectors. The data used comes from the Chinese web page text data of Wikipedia in April 2018. The CBOW model is adopted, and the word vector dimension is 300 dimensions. Subsequent deep learning models are based on the word vectors completed by the training;
[0029] Manual construction of question data R in the field of travel 1 Article, using a custom query to crawl the question data R in the travel field 2 Article, to obtain the question data R of the chat class and the ve...
specific Embodiment approach 3
[0038] Specific implementation mode three: combination image 3 Describe this embodiment. This embodiment further defines a human-machine multi-round dialogue method for the travel field described in Embodiment 1; the CNN model is used to obtain the intention information of the current question after step 1 processing. The specific process is:
[0039] Manual construction of question data R in the field of travel 1 Article, using a custom query to crawl the question data R in the travel field 2 Article, to obtain the question data R of the chat class and the vertical class in the SMP2017 evaluation task 3 strip; put all R 1 +R 2 +R 3 The questions are randomly sorted, and after random sorting, a part of the questions are randomly selected as the training set, and the rest of the questions are used as the test set; the CNN model includes an input layer, a convolutional layer, a pooling layer and a fully connected layer;
[0040] The training process of the CNN model is as...
PUM
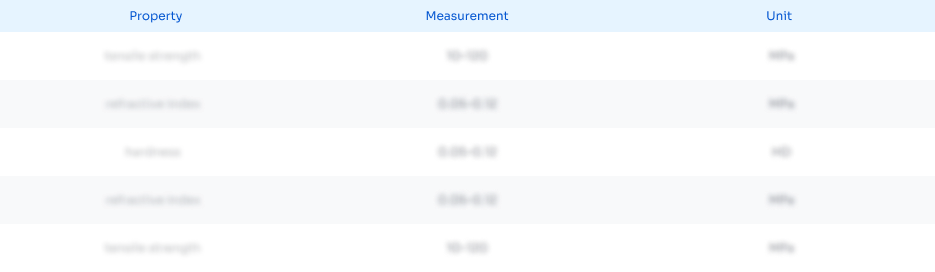
Abstract
Description
Claims
Application Information
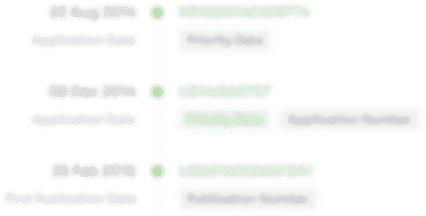
- R&D Engineer
- R&D Manager
- IP Professional
- Industry Leading Data Capabilities
- Powerful AI technology
- Patent DNA Extraction
Browse by: Latest US Patents, China's latest patents, Technical Efficacy Thesaurus, Application Domain, Technology Topic, Popular Technical Reports.
© 2024 PatSnap. All rights reserved.Legal|Privacy policy|Modern Slavery Act Transparency Statement|Sitemap|About US| Contact US: help@patsnap.com