Tensor structural missing filling method based on joint low rank and sparse representation
A technology of sparse representation and filling method, applied in the field of computer vision, which can solve problems such as inability to effectively recover tensor data
- Summary
- Abstract
- Description
- Claims
- Application Information
AI Technical Summary
Problems solved by technology
Method used
Image
Examples
Embodiment Construction
[0055] The present invention aims to make up for the deficiency of the prior art, that is, to realize accurate filling of structurally missing tensors. The technical scheme adopted by the present invention is a tensor filling method with structural loss based on TT low-rank tensor filling and fiber signal sparse representation, the steps are, based on TT low-rank tensor filling theory, introducing TT low-rank prior pair At the same time, considering that the fiber signal along each dimension of the tensor can be sparsely represented by the dictionary, and the missing fiber in the previous dimension can be restored by sparsely constraining the fiber signal in the next dimension, so the The fiber signal of each dimension introduces sparse constraints; based on the above-mentioned joint TT low-rank and sparse priors of each dimension, the tensor filling problem with missing structure is specifically formulated as a constrained optimization problem, so as to realize the Sexually m...
PUM
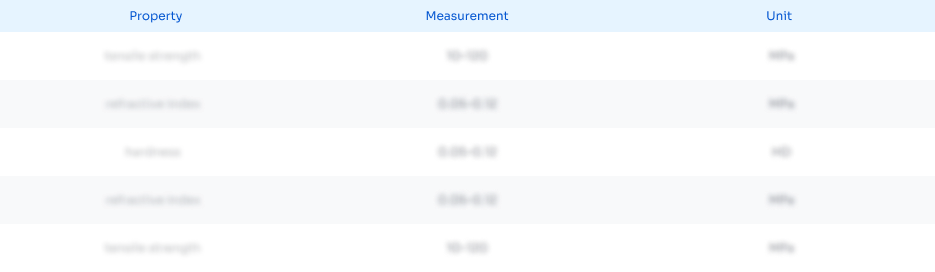
Abstract
Description
Claims
Application Information
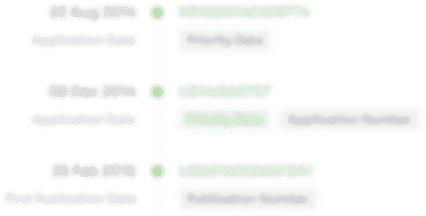
- R&D Engineer
- R&D Manager
- IP Professional
- Industry Leading Data Capabilities
- Powerful AI technology
- Patent DNA Extraction
Browse by: Latest US Patents, China's latest patents, Technical Efficacy Thesaurus, Application Domain, Technology Topic, Popular Technical Reports.
© 2024 PatSnap. All rights reserved.Legal|Privacy policy|Modern Slavery Act Transparency Statement|Sitemap|About US| Contact US: help@patsnap.com