A hyperspectral remote sensing data classification method based on depth neural network
A deep neural network and hyperspectral remote sensing technology, applied in the field of hyperspectral data supervision and classification, can solve the problem of a small number of labeled samples, and achieve the effect of improving generalization performance and avoiding the loss of detailed information.
- Summary
- Abstract
- Description
- Claims
- Application Information
AI Technical Summary
Problems solved by technology
Method used
Image
Examples
Embodiment Construction
[0024] In order to better illustrate the hyperspectral remote sensing data classification method involved in the present invention, AVIRIS hyperspectral data is used for fine classification. A method for classifying hyperspectral remote sensing data based on a deep neural network of the present invention, the specific implementation steps are as follows:
[0025] (1) Read in hyperspectral data: the hyperspectral image of the Salinas Valley with a size of 512×217 is used as the source domain data, and the hyperspectral image of the Indiana pine forest with a size of 145×145 is used as the target domain data. The data are all acquired by AVIRIS, and the band interval is 370nm-2507nm, all of which contain 220 bands and have the same wavelength information;
[0026] (2) Determine the number of categories, and select training samples and test samples: where the number of classification categories in the source domain is J 1 =16, the number of classification categories in the targe...
PUM
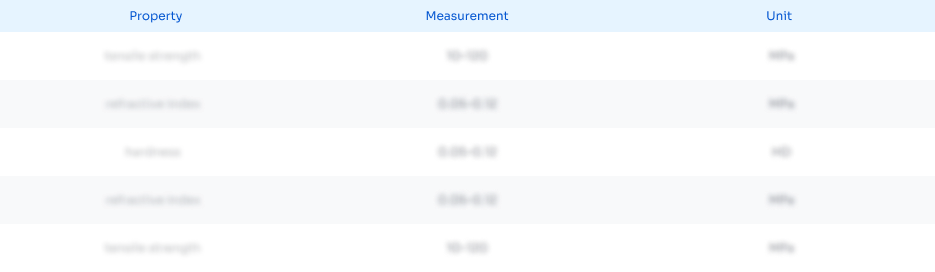
Abstract
Description
Claims
Application Information
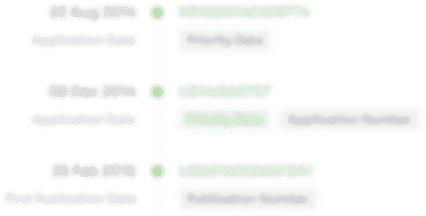
- R&D Engineer
- R&D Manager
- IP Professional
- Industry Leading Data Capabilities
- Powerful AI technology
- Patent DNA Extraction
Browse by: Latest US Patents, China's latest patents, Technical Efficacy Thesaurus, Application Domain, Technology Topic, Popular Technical Reports.
© 2024 PatSnap. All rights reserved.Legal|Privacy policy|Modern Slavery Act Transparency Statement|Sitemap|About US| Contact US: help@patsnap.com