Video classification based on hybrid convolution and attention mechanism
A video classification and attention technology, applied in the field of image processing, can solve the problems of large amount of calculation in end-to-end training dual-stream network, difficult to determine the local position of video frames, and no great improvement, etc., to reduce computational complexity, The effect of reducing model complexity and improving accuracy
- Summary
- Abstract
- Description
- Claims
- Application Information
AI Technical Summary
Problems solved by technology
Method used
Image
Examples
Embodiment 1
[0033] With the popularity of short videos, people's research has moved from the field of images to the field of video, and there is also a great demand for video classification. The shortcomings of existing technologies in solving video classification problems lie in low accuracy and poor real-time performance. For this reason, the present invention proposes a video classification method based on hybrid convolution and attention mechanism through research and innovation, see figure 1 , the present invention utilizes the spatial information and time information corresponding to the video to carry out spatio-temporal feature extraction and adopts an end-to-end strategy to classify the video, including the following steps:
[0034] (1) Select a video classification dataset: first select the corresponding dataset for the video to be classified and input it. For example, when classifying human action videos, input the human action video dataset, and all the input datasets are used ...
Embodiment 2
[0047] The video classification method based on mixed convolution and attention mechanism is the same as embodiment 1, and obtains the video mixed convolution feature map described in the step (5) of the present invention in the direction of the time series dimension, including the following steps:
[0048] (5a) Obtain the hybrid convolutional feature maps of two video clips: input the preprocessed two video clips into the constructed hybrid convolutional neural network, and obtain the final result of the two input video clips on the hybrid convolutional neural network. 2048 5×5 pixel feature maps output by a convolutional layer conv.
[0049] (5b) Merge the mixed convolution feature maps of the two video clips in the direction of the time sequence dimension to obtain the video mixed convolution feature map: 2048 5×5 pixel convolution feature maps of the two input video clips in the time sequence Combined in the dimension direction, 2048 mixed convolution feature maps of 5×5 p...
Embodiment 3
[0052] The video classification method based on mixed convolution and attention mechanism is the same as embodiment 1-2, and obtains video attention feature map with attention mechanism operation described in the step (6) of the present invention, carries out as follows:
[0053] (6a) The shape of the obtained video hybrid convolution feature map is expressed as 2048×2×5×5, where 2048 is the number of channels, 2 is the timing length, and two 5s are the height and width of the video hybrid convolution feature map, respectively.
[0054] (6b) The video mixed convolution feature map is expanded into 2048 feature vectors, and the feature vector dimension is 2×5×5=50, forming a feature vector matrix with a size of 2048×50.
[0055] (6c) Calculate the eigenvector matrix F 1 and F 2 The inner product of is carried out according to the following formula:
[0056]
[0057] Among them, the eigenvector matrix F 1 is the original matrix, the eigenvector matrix F 2 for F 1 The tra...
PUM
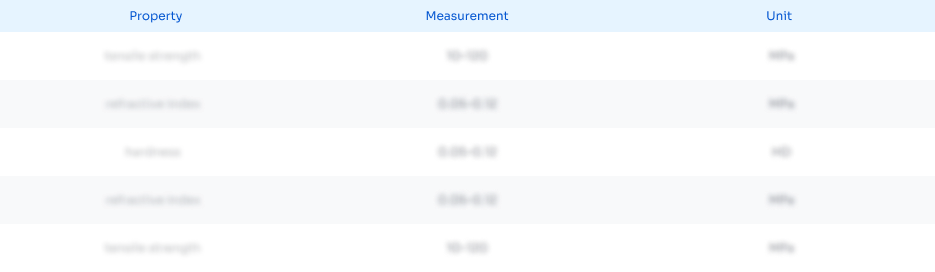
Abstract
Description
Claims
Application Information
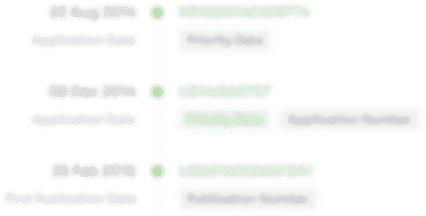
- R&D Engineer
- R&D Manager
- IP Professional
- Industry Leading Data Capabilities
- Powerful AI technology
- Patent DNA Extraction
Browse by: Latest US Patents, China's latest patents, Technical Efficacy Thesaurus, Application Domain, Technology Topic, Popular Technical Reports.
© 2024 PatSnap. All rights reserved.Legal|Privacy policy|Modern Slavery Act Transparency Statement|Sitemap|About US| Contact US: help@patsnap.com