A angle-image multi-stage neural network based 3D reconstruction method
A neural network and three-dimensional reconstruction technology, applied in the field of computer vision, can solve problems such as difficulty in fully mining image visual clues, large differences in three-dimensional shapes and shapes, and limited learning ability of a single neural network.
- Summary
- Abstract
- Description
- Claims
- Application Information
AI Technical Summary
Problems solved by technology
Method used
Image
Examples
Embodiment
[0148] In this example, if figure 2 Shown is the input image to be reconstructed, through the three-dimensional reconstruction method of the present invention, the three-dimensional shape of the object in the picture can be reconstructed. The specific implementation process is as follows:
[0149] Through steps 1 to 4, the present invention obtains a trained point cloud generation network model and a point cloud refinement network model, the former is used to generate initial point clouds, and the latter is used to generate fine point clouds.
[0150] In step five, the user inputs an image containing the chair object to be reconstructed, such as figure 2 shown. The image is input into the point cloud generation network model, and is encoded into the image information feature matrix by the image encoder composed of the deep residual network. This feature matrix is then fed into the primary decoder, where the deconvolution branch of the decoder maps the feature matrix int...
PUM
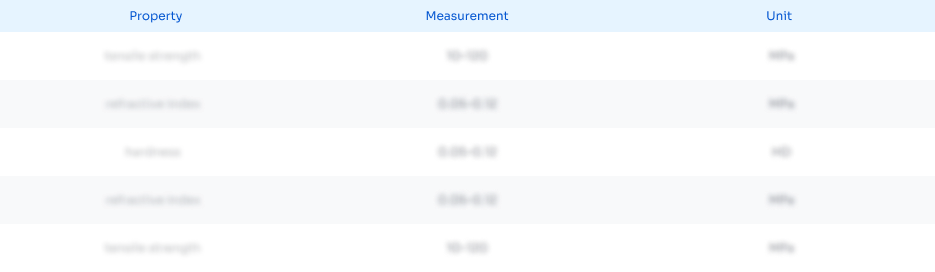
Abstract
Description
Claims
Application Information
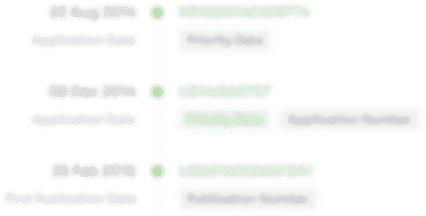
- R&D Engineer
- R&D Manager
- IP Professional
- Industry Leading Data Capabilities
- Powerful AI technology
- Patent DNA Extraction
Browse by: Latest US Patents, China's latest patents, Technical Efficacy Thesaurus, Application Domain, Technology Topic, Popular Technical Reports.
© 2024 PatSnap. All rights reserved.Legal|Privacy policy|Modern Slavery Act Transparency Statement|Sitemap|About US| Contact US: help@patsnap.com