A road environment visual perception method based on an improved Faster R-CNN
A technology of visual perception and road environment, which is applied in the direction of instruments, character and pattern recognition, scene recognition, etc., can solve the safety and reliability constraints of autonomous driving technology, the promotion and popularization of driverless cars, the wrong prediction of targets, and the inaccurate characteristics Improve the generalization ability and detection accuracy, reduce the missed detection rate, and improve the detection ability
- Summary
- Abstract
- Description
- Claims
- Application Information
AI Technical Summary
Problems solved by technology
Method used
Image
Examples
Embodiment Construction
[0037] In order to describe in detail the technical content, structural features, achieved goals and effects of the technical solution, the following will be described in conjunction with specific embodiments and accompanying drawings.
[0038] The present invention proposes a road environment visual perception method based on improved Faster R-CNN, which comprises the following steps:
[0039] S1. Before the input image enters the network model, it is first scaled to 1600*700, and then enters the ResNet-101 feature extraction network in the Featureextraction network module, such as figure 2 shown. After the Conv1, Conv2_x, Conv3_x, and Conv4_x of ResNet-101 have a total of 91 layers of fully convolutional networks, the Feature maps of the pictures are extracted;
[0040] S2. The Feature maps output by the Feature extraction network module enter the Regionproposal network module, such as figure 1 shown. The Region proposal network module uses a 3*3 sliding window to traver...
PUM
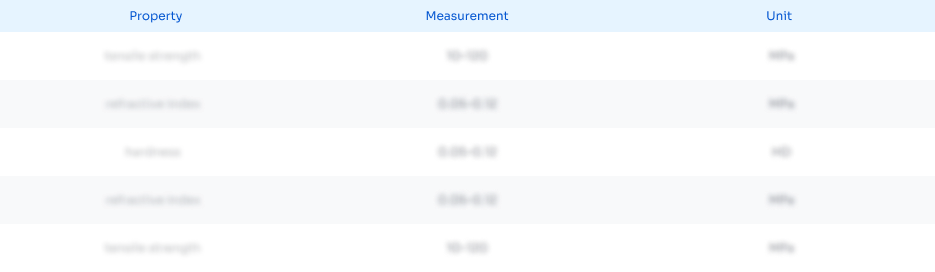
Abstract
Description
Claims
Application Information
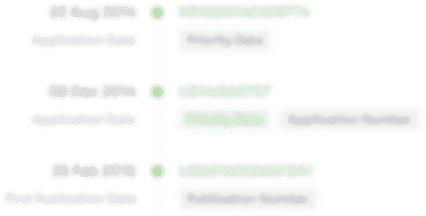
- Generate Ideas
- Intellectual Property
- Life Sciences
- Materials
- Tech Scout
- Unparalleled Data Quality
- Higher Quality Content
- 60% Fewer Hallucinations
Browse by: Latest US Patents, China's latest patents, Technical Efficacy Thesaurus, Application Domain, Technology Topic, Popular Technical Reports.
© 2025 PatSnap. All rights reserved.Legal|Privacy policy|Modern Slavery Act Transparency Statement|Sitemap|About US| Contact US: help@patsnap.com