A Dynamically Reconfigurable Convolutional Neural Network Accelerator Architecture for the Internet of Things
A convolutional neural network and Internet of Things technology, applied in the field of dynamic reconfigurable convolutional neural network accelerator architecture, can solve the problems of energy efficiency (low performance/power consumption, inability to apply smart mobile terminals, high power consumption, etc.) The effects of external memory access, simple network structure, and low power consumption
- Summary
- Abstract
- Description
- Claims
- Application Information
AI Technical Summary
Problems solved by technology
Method used
Image
Examples
Embodiment
[0090] Regarding the speed index, the superiority of the present invention comes from the design of the processing unit array and cache architecture. First, the processing unit adopts the Winograd convolution acceleration algorithm. For example, for a convolution operation with a size of 5*5 input data, a convolution kernel size of 3*3, and a step size of 1, traditional convolution needs to introduce 81 multiplication operations, while this It is published that each processing unit only needs to introduce 25 multiplications. In addition, the processing unit array is in the convolutional network, and the input channel and the output channel are processed in a certain degree of parallelism, which makes the convolution operation faster. On the other hand, the cache architecture has two working modes. In the on-chip working mode, the data generated by the middle layer of the convolutional neural network does not need to be stored off-chip, but can be directly sent to the next layer...
PUM
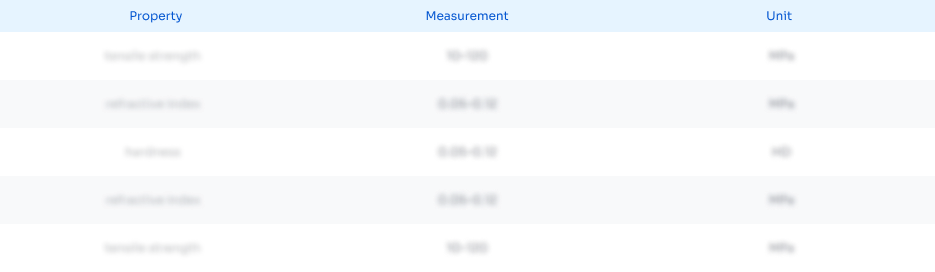
Abstract
Description
Claims
Application Information
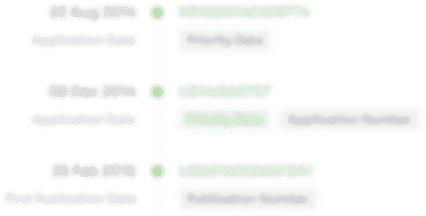
- R&D Engineer
- R&D Manager
- IP Professional
- Industry Leading Data Capabilities
- Powerful AI technology
- Patent DNA Extraction
Browse by: Latest US Patents, China's latest patents, Technical Efficacy Thesaurus, Application Domain, Technology Topic, Popular Technical Reports.
© 2024 PatSnap. All rights reserved.Legal|Privacy policy|Modern Slavery Act Transparency Statement|Sitemap|About US| Contact US: help@patsnap.com