Dynamic industrial process fault diagnosis method based on GRU depth neural network
A deep neural network, industrial process technology, applied in the field of dynamic industrial process fault diagnosis, can solve problems such as aggravating the disaster of dimensionality and instability of feature extraction methods
- Summary
- Abstract
- Description
- Claims
- Application Information
AI Technical Summary
Problems solved by technology
Method used
Image
Examples
Embodiment Construction
[0042] Example figure 1 Shown, the present invention is based on the dynamic industrial process fault diagnosis method of GRU deep neural network and comprises the following steps:
[0043] Step 1. Suppose there are n groups of original experimental data X=[x 1 ,x 2 ...x n ], Set the time step T as 3, use the moving window to preprocess the original experimental data, and divide the original n groups of experimental data into [x 1 ,x 2 ,x 3 ], [x 2 ,x 3 ,x 4 ], [x 3 ,x 4 ,x 5 ] sequence unit, there are n-T sequence units;
[0044] in, Indicates the dimension of each group of original experimental data x, is a mathematical symbol, representing the real phasor space, d x Represents the characteristic number of x;
[0045] Step 2, the sequence unit X (1) =[x 1 ,x 2 ,x 3 ], X (2) =[x 2 ,x 3 ,x 4 ]…X (m) =[x n-2 ,x n-1 ,x n ] input to the GRU deep neural network optimized by the batch normalization algorithm, each input sequence unit X (i) Obtain the...
PUM
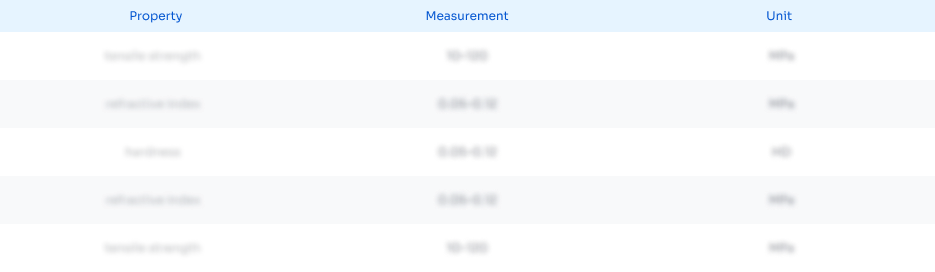
Abstract
Description
Claims
Application Information
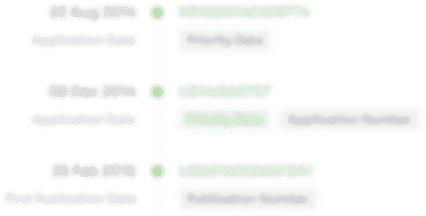
- R&D Engineer
- R&D Manager
- IP Professional
- Industry Leading Data Capabilities
- Powerful AI technology
- Patent DNA Extraction
Browse by: Latest US Patents, China's latest patents, Technical Efficacy Thesaurus, Application Domain, Technology Topic, Popular Technical Reports.
© 2024 PatSnap. All rights reserved.Legal|Privacy policy|Modern Slavery Act Transparency Statement|Sitemap|About US| Contact US: help@patsnap.com