Bearing fault degree identifying method based on flow diagram and non-nave Bayesian inference
An identification method and technology of failure degree, applied in mechanical bearing testing, mechanical component testing, machine/structural component testing, etc., can solve the problems of low diagnostic accuracy, weak classification and reasoning ability, high computational cost, etc. Computational complexity, overcoming conditional constraints, the effect of good diagnostic results
- Summary
- Abstract
- Description
- Claims
- Application Information
AI Technical Summary
Problems solved by technology
Method used
Image
Examples
specific Embodiment approach 1
[0024] Specific implementation mode one: combine figure 1 This embodiment will be described. This embodiment provides a bearing fault degree identification method based on flow diagram and non-naive Bayesian reasoning, the method includes the following steps:
[0025] Step 1. Extract the fault diagnosis features of the roller bearings in the training samples to construct a standardized flow diagram;
[0026] Step 2, using the node reduction algorithm based on the importance of symptom attribute nodes to delete redundant symptom attribute nodes in the standardized flow graph, and obtain the node-reduced flow graph;
[0027] Step 3: Extract the fault diagnosis features of the roller bearings in the waiting samples, and use the non-naive Bayesian inference algorithm based on the flow graph to identify the state of the roller bearings in the waiting samples.
specific Embodiment approach 2
[0028] Specific implementation mode two: combination figure 2 This embodiment will be described. The difference between this embodiment and the specific embodiment one is: the specific steps of the node reduction algorithm based on the importance of symptom attribute nodes in the second step are as follows:
[0029] Step 21, calculating the information entropy H(G) of the standardized flow graph G;
[0030] Step 22: Calculate symptom attribute node set N C Relative to the decision attribute node set N D The mutual information H(N C ,N D );
[0031] Step two and three, calculate symptom attribute node x i ∈N C ,(i=1,...,m), m is the number of symptom attribute nodes, Sig(x i ,N D ) is relative to the decision attribute node set N D the importance of
[0032] Step 24, if symptom attribute node x i ∈N C The importance of Sig(x i ,N D )=0, then symptom attribute node x i is unnecessary, otherwise essential;
[0033] Step 25, repeat steps 23 and 24 for other sympt...
specific Embodiment approach 3
[0035] Specific embodiment three: the difference between this embodiment and specific embodiment two is: the symptom attribute node x∈N in the step two or three C Relative to the decision attribute node set N D The importance of Sig(x,N D ) is defined as follows:
[0036] Let the standardized flow graph G=(N,B,σ), N represents the node set, B represents the directed branch set, σ is the standardized flow function, N C and N D are symptom attribute node set and decision attribute node set respectively, then symptom attribute node x∈N C Relative to the decision attribute node set N D The importance is:
[0037] Sig(x,N D )=H(N C ,N D )-H(N C -{x},N D ) (1).
[0038] Among them, H(N C ,N D ) is symptom attribute node set N C Relative to the decision attribute node set N D The mutual information of H(N C -{x},N D ) is to remove symptom attribute node x∈N C Post symptom attribute node set N C - {x} relative to decision attribute node set N D mutual information. ...
PUM
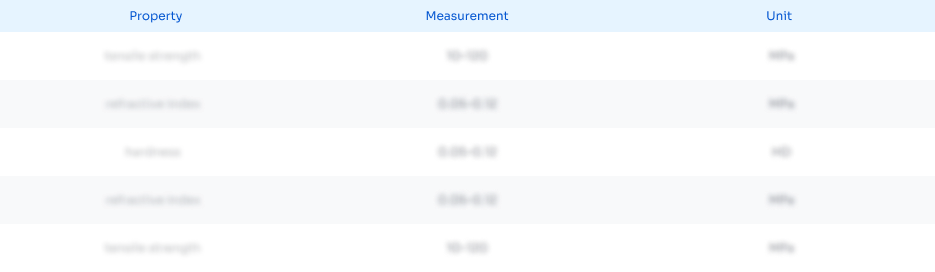
Abstract
Description
Claims
Application Information
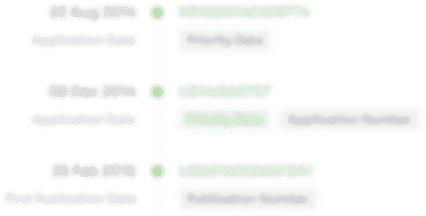
- R&D Engineer
- R&D Manager
- IP Professional
- Industry Leading Data Capabilities
- Powerful AI technology
- Patent DNA Extraction
Browse by: Latest US Patents, China's latest patents, Technical Efficacy Thesaurus, Application Domain, Technology Topic.
© 2024 PatSnap. All rights reserved.Legal|Privacy policy|Modern Slavery Act Transparency Statement|Sitemap