A triple-optimal semi-supervised regression algorithm based on a self-training framework
A regression algorithm and self-training technology, applied in computing, computer parts, instruments, etc., can solve the problem of less cost of obtaining label samples
- Summary
- Abstract
- Description
- Claims
- Application Information
AI Technical Summary
Problems solved by technology
Method used
Image
Examples
Embodiment Construction
[0023] Combine below figure 1 Shown, the present invention is described in further detail:
[0024] A three-optimized semi-supervised regression algorithm under a self-training framework, comprising the following steps:
[0025] Step 1: Screen unlabeled samples and labeled samples, and use the filtered labeled samples to establish a Gaussian process regression model f 1 , use the model to predict the unlabeled sample set M 1 label value to get the pseudo-label sample set S 1 ;
[0026] Unlabeled sample screening: given a threshold θ 1 , using the Mahalanobis distance to measure the unlabeled sample x′ i The similarity d with the center C of the labeled sample dense area i , if x′ i The distance from C is less than θ 1 , then x′ i Satisfy the preferred conditions;
[0027] Labeled sample screening: given a threshold θ 2 , using the Mahalanobis distance to measure the similarity d(x i ,x j ), statistical sample x i with surrounding samples x j The Mahalanobis dist...
PUM
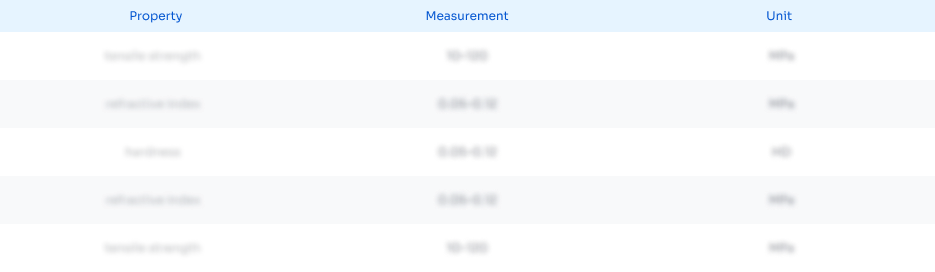
Abstract
Description
Claims
Application Information
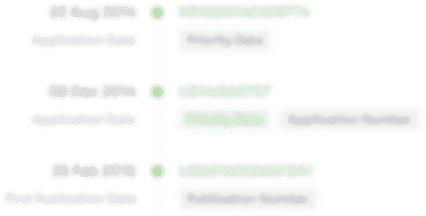
- R&D Engineer
- R&D Manager
- IP Professional
- Industry Leading Data Capabilities
- Powerful AI technology
- Patent DNA Extraction
Browse by: Latest US Patents, China's latest patents, Technical Efficacy Thesaurus, Application Domain, Technology Topic, Popular Technical Reports.
© 2024 PatSnap. All rights reserved.Legal|Privacy policy|Modern Slavery Act Transparency Statement|Sitemap|About US| Contact US: help@patsnap.com