A Raman spectroscopic analysis method based on a convolution neural network
A convolutional neural network and Raman spectroscopy technology, applied in neural learning methods, biological neural network models, neural architectures, etc., can solve problems such as limit extraction, noise and baseline drift, cumbersome denoising and baseline correction, and achieve interpretation Strong, easy-to-monitor effects
- Summary
- Abstract
- Description
- Claims
- Application Information
AI Technical Summary
Problems solved by technology
Method used
Image
Examples
Embodiment 1
[0100] Example 1: Application of the present invention to the analysis of blood samples.
[0101] A total of 326 training samples were used in the experiment, 110 human blood samples were collected from Chongqing Southwest Hospital, and 216 animal blood samples were collected from Chongqing Academy of Traditional Chinese Medicine. Compared with the traditional methods PLS-DA and SVM, the classification accuracy is increased by 3.67% and 4.59%, respectively.
[0102] The following is an introduction to the specific RS-CNN.
[0103] The output of the convolutional denoising layer C1 in RS-CNN is as follows Figure 5 As shown (the normalized result is shown in the figure for easy comparison), the denoising effect is obvious. Figure 6 The convolutional denoising layer layer C1 convolution kernel coefficients are given. The convolution kernel of the convolutional denoising layer C1 should not be too large, which will lead to the loss of spectral peak information and affect the ...
PUM
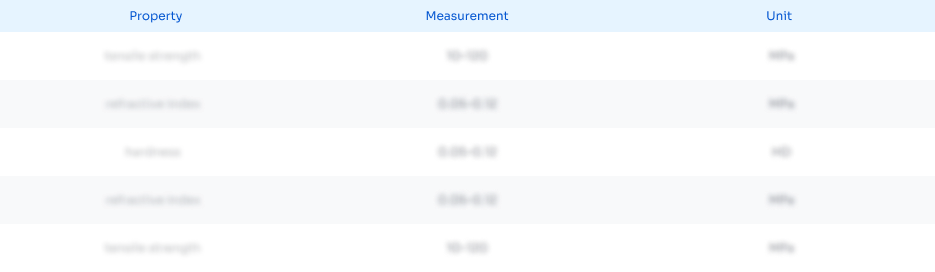
Abstract
Description
Claims
Application Information
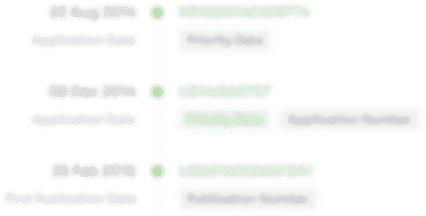
- R&D Engineer
- R&D Manager
- IP Professional
- Industry Leading Data Capabilities
- Powerful AI technology
- Patent DNA Extraction
Browse by: Latest US Patents, China's latest patents, Technical Efficacy Thesaurus, Application Domain, Technology Topic, Popular Technical Reports.
© 2024 PatSnap. All rights reserved.Legal|Privacy policy|Modern Slavery Act Transparency Statement|Sitemap|About US| Contact US: help@patsnap.com