A hyperspectral classification method based on attention constraint non-negative matrix factorization
A non-negative matrix decomposition and hyperspectral classification technology, applied in the field of hyperspectral classification, can solve the problems of low classification accuracy of hyperspectral images, difficulty in hyperspectral recognition and classification, and misclassification of ground object types, so as to improve classification accuracy and convergence speed up effect
- Summary
- Abstract
- Description
- Claims
- Application Information
AI Technical Summary
Problems solved by technology
Method used
Image
Examples
Embodiment Construction
[0043] The accompanying drawings are for illustrative purposes only and cannot be construed as limiting the patent;
[0044] In order to better illustrate this embodiment, some parts in the drawings will be omitted, enlarged or reduced, and do not represent the size of the actual product;
[0045] For those skilled in the art, it is understandable that some well-known structures and descriptions thereof may be omitted in the drawings.
[0046] The technical solutions of the present invention will be further described below in conjunction with the accompanying drawings and embodiments.
[0047] This embodiment provides a hyperspectral classification method based on attention-constrained non-negative matrix factorization, which specifically includes the following steps:
[0048] S1: First read a hyperspectral image, the known number of categories is r, and get the hyperspectral image matrix X=[x 1 ,...,x m ] T∈ R m×n ; X is an m-dimensional matrix.
[0049] Among them, m r...
PUM
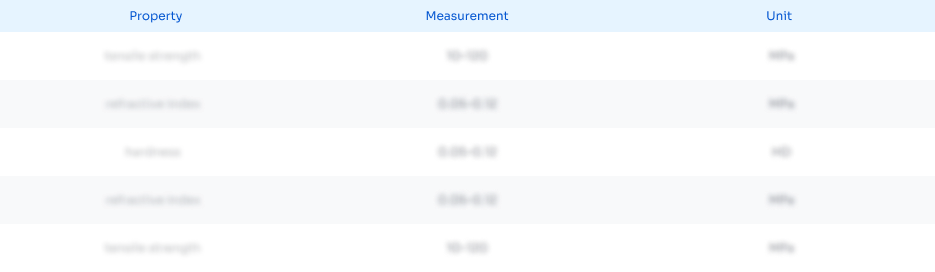
Abstract
Description
Claims
Application Information
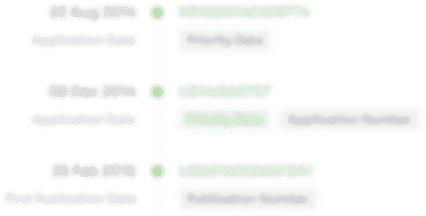
- R&D Engineer
- R&D Manager
- IP Professional
- Industry Leading Data Capabilities
- Powerful AI technology
- Patent DNA Extraction
Browse by: Latest US Patents, China's latest patents, Technical Efficacy Thesaurus, Application Domain, Technology Topic, Popular Technical Reports.
© 2024 PatSnap. All rights reserved.Legal|Privacy policy|Modern Slavery Act Transparency Statement|Sitemap|About US| Contact US: help@patsnap.com